What is Long-Thinking AI and Why Does it Matter?
Beyond speed now lies depth. Welcome Long-thinking AI. A paradigm shift from speedy responses to logical reasoning. It uses advanced techniques to minimize errors and offers vast potential across various fields. So uncover the capabilities of reasoning AI along with the challenges it holds.
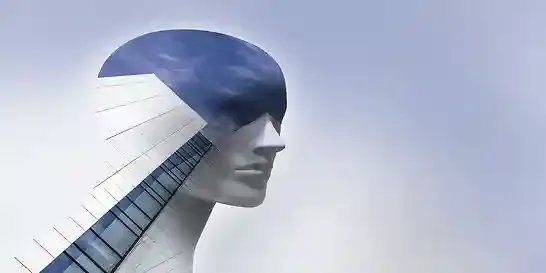
For the past few decades, the AI field has long been focused on creating systems that accomplish tasks by using human intelligence. Initially, the core focus was primarily on speed in generating information and computation completions. However, now the complexity of real-world problems requires more up-to-date solutions. So we can say that a significant shift is underway. This shift is towards AI, which can prioritize depth, logic, and reasoning regarding the problem rather than merely speedy responses. This engaging paradigm shift is called Long-thinking AI.
With this new technology, we can say that AI has become one of the most exciting tech trends of the 21st century. Its market size is increasing day by day. As per Statista, in 2025, the AI market size is amounting near 244 billion U.S. dollars. With every new tech innovation just like long-thinking AI, the market is expected to grow to over 800 billion U.S. dollars by 2030. So, it's obvious how much technology is budding day by day. Deep-thinking AI is very essential for those challenges which require a lot of extended reasoning and understanding. So, this paradigm shift holds the potential to address the people's most intricate challenges. Having said that, the article explores all the possible understandings behind the term long-thinking AI, its applications, and possible future challenges.
Defining Long-Thinking AI: Core Principles
Long-thinking AI distinguishes itself from conventional AI approaches. Unlike speedy responses and quick answers, it focuses on detailed and logical methodology for problem-solving and well-structured outputs. The major objective of advanced-reasoning AI is to diminish the possibility of hallucinations, where AI models tend to generate nonsensical information.
Moreover, long-thinking AI contains reflective computation. It enables the AI systems to first engage with data thoughtfully before concluding. This characteristic is a true contradiction with short-thinking AI models where the earlier LLMs often focus on rapid answers. There's no doubt that the short-thinking AI models have shown remarkable capabilities in multiple tasks. However, they often disappoint the users with highly multifaceted problems that require deep reasoning and understanding of context.
So, the building of AI systems involves the exchange between the speed and the accuracy. In the case of long-thinking AI, the focus is on accuracy and depth for better results in terms of specific applications.
Technical Foundations of Long-Thinking AI
Technically, long-thinking AI uses innovative deep learning algorithms, like transformer architecture and LLMs to identify complicated patterns and generate responses. Along with these neural network-based approaches, long-thinking AI often incorporates symbolic AI. This AI approach helps in more knowledge-based problem-solving.
So, by combining the strengths of both knowledge and symbolic-based reasoning, long-thinking AI aims to create more logic-based AI systems with fewer errors.
Furthermore, the long context window utilization is a crucial part of long-thinking AI. It basically allows the AI models to retain and process large amounts of info within a single session. So, this expanded memory feature allows this AI to deal with broader contexts while maintaining the lucidity between prolonged interactions.
Long-Thinking AI Example: Anthropic’s Claude and Visible Thinking
A notable example nowadays of the application of long-thinking AI is the development of the Anthropic Model; Claude 3.7 Sonnet. This model has a feature which is “Extended Thinking Mode”. Users can activate this feature to direct the system toward the more profound analysis of complex queries. Even with this feature, the developers can set a "Thinking Budget”, which specifies the time and computational resource that Claude can assign to the specific problem. This extraordinary capability allows the core models to put more exertion on reasoning. This whole process potentially leads to a substantial increase in intelligence for the most thought-provoking tasks.
Apart from that, Anthropic has also discovered making the whole Claude thought process visible. When this mode is enabled, users can see how AI reasons. By doing so, it also offers potential benefits in terms of user trust and model configuration as well. However, this particular visibility also raises questions about the potential misuse of the model's internet state.
Potential Applications of Long-Thinking AI across Various Domains
The ability of long-thinking AI to solve complex problems with logical reasoning opens up a wide array of applications across various domains which are as follows;
Science and Research
In terms of science and research, long-thinking AI can be so helpful in solving complex mathematical problems which require long logical reasoning. Secondly, it can also help in accelerating the scientific discovery process. This can be done through generating detailed research proposals and creating innovative hypotheses. So, by analyzing enormous datasets through the analysis of context, critical-thinking AI can truly identify the eloquent trends and patterns that might avoid human researchers.
Moreover, its ability to gather and synthesize information simultaneously from multiple sources makes it a powerful tool for research.
Healthcare
Like science and research, in healthcare long-thinking AI also holds the potential to upgrade multiple medical practices. It can assist in the drug discovery process by discovering a gathering of molecular combainations and stimulating their latent effects for a large period. Moreover, long-thinking AI can also help in developing highly efficient care plans for patients. It can be done by analyzing the comprehensive data of the patients. Data could be medical history, genetic information, and several treatment responses.
Furthermore, its ability to process patient records and medical literature helps in improving diagnostic accuracy.
Financial Industry
Long-thinking AI can highly benefit the financial industry as well. This technology has the capability to tackle and analyze long-form documents such as loan agreements, market research reports, etc. it leads to more accurate and insightful decision making. On the other hand, long thinking AI has also the ability to process extensive transaction data and address some anomalies to make the system less fraudulent and risky.
Furthermore, it can also be applied to algorithmic trading to enable the processing of unstructured data into more informed trading strategies. In terms of personalization, long-thinking AI can also be valuable for analyzing individual financial circumstances to provide tailored personalized advice, thus making financial planning more accessible to a large audience.
Advanced AI Agents and Assistants
The increase of advanced AI agents is yet another application to look forward to in terms of long-thinking AI. These personalized beings can maintain the context across various lengthy conversations, leading toward more natural collaborations. Moreover, long-thinking AI allows AI agents to complete difficult tasks by reacting dynamically to changes in their environment. This capacity allows the AI systems to truly assist users with multi-layered needs.
Long-Thinking AI: Comparison with Traditional and Generative AI
To better understand the concept of long-thinking AI, it would be more helpful to make a comparison with traditional and generative AI for better understanding;
Traditional AI
Traditional AI is often referred to as narrow AI. It typically depends on the rule-based system and algorithms intended for detailed tasks. In terms of training, these systems often depend upon categorized datasets. This process is typically known as supervised learning.
Traditional AI usually surpasses;
- Pattern recognition
- Automation
- Predictive analytics
- Providing predictable results and offering greater transparency.
However, it has limited capabilities when things go outside of its specific training.
Generative AI
In contrast, generative AI mainly focuses on generating new and original content. Content like images, text, music, videos and, more. Generative AI models often act on existing data but with unsupervised learning methods. While these models are very powerful in terms of content creation, hypothesis generation, and designs GenAI models often function as black boxes. It means that the decision-making processes are less transparent. Apart from that, they also require a high amount of computational power and resources to train large datasets.
Evolutionary Long-Thinking AI
Apart from traditional and GenAI, long-thinking AI can be seen as an evolution. It builds upon the basis of deep learning by placing great stress on reasoning and accuracy for complex problems. More logical approaches often incorporate the elements of symbolic AI. The primary focus of long-thinking AI is to tackle such tasks which require multi-step reasoning. In this sense, long-thinking AI aims to overcome the limitations of both traditional and generative AI to handle complicated real-world problems.
Comparison Table
Features | Traditional AI (Analytical/Narrow AI) | Generative AI (GenAI) | Long Thinking AI |
---|---|---|---|
Approach | Rule-based, algorithmic | Content generation | Logical, detailed, analytical reasoning |
Learning Method | Supervised (labeled data) | Often Unsupervised (existing data) | Deep learning, often hybrid with symbolic AI |
Focus | Automation, prediction, classification | Creating new content | Accuracy, deep reasoning, complex problem-solving |
Adaptability | Limited to specific tasks | Adaptable across domains for content creation | Designed for complex, novel challenges |
Transparency | Generally high | Often low ("black box") | Aiming for increased transparency (e.g., Claude) |
Computational Resources | Generally lower | Often very high | Very high |
Typical Applications | Automation, customer support, fraud detection | Content creation, art, text generation, and research | Scientific discovery, complex planning, healthcare diagnosis |
Key Challenges and Considerations
While long thinking AI offers promising solutions and presents a promising path forward. Its development and application are escorted by some challenges which are as follows;
Computational Resources
One of the most noteworthy challenges is the considerable computational resources that are required to power these systems. The energy consumption associated with long-thinking AI can potentially lead to unfavorable environmental impact due to the augmented demands of data centers. However, from a practical point of view, the high computing cost may also create some sort of financial barrier. This could create difficulty for small businesses and research institutions to fully engage in the consumption of long-thinking AI solutions.
Technical Challenges
Another key technical challenge is the hazard of overfitting. As we know, long-thinking AI depends on multifaceted architectures with billions of parameters. Through these complex structures long thinking AI becomes capable of sophisticated decision making. But at the same, it also increases the risks of the model memorizing the training data rather than simplifying to unseen or new situations. So, ensuring the accuracy of the model across wider inputs is a critical area of research.
User Experience (UX)
User experience also presents a potential challenge in long-thinking AI evolution. The features for reasoning customization like thinking budgets must be really confusing for new users. So the developer will need to design the interfaces very carefully to cater to both amateur and expert users.
Practical and Ethical Hurdles
Beyond technical considerations, there are some ethical hurdles as well which need to be overcome. Marinating the availability of fresh data is very important. Old data can lead to potentially inaccurate or irrelevant outputs. Another significant concern is that the over-reliance on long-thinking AI could lead to diminished human thinking skills. Like if humans become very accustomed to pre-ready complex AI-generated solutions then there is a risk that their reasoning skills may weaken with time.
Ethical considerations are very essential in the development of long-thinking AI. As we know these systems are based on large data sets which may contain some biases. So ensuring fairness and accountability is very crucial, particularly in domains like healthcare and finance.
Long Thinking AI and the Future: Final Thoughts
In conclusion, long-thinking AI represents a remarkable evolution in the field. It is ready to shift the focus from rapid completion of tasks to slow but in-depth analysis. Drawing inspiration from human deliberative thoughts, Advanced reasoning AI emphasizes a detailed and analytical approach. The ongoing research efforts powered by long-thinking AI aim to explore advanced reasoning techniques to further enhance the competencies of the systems.
However, the developments and implementations of the long thinking AI also came up with significant hurdles. The ultimate risk of overfitting, substantial computational costs, and the need for user-friendly interfaces are some challenges that must be addressed.
Looking Ahead, long-thinking AI has the potential to reshape society with innovation and efficiency. While distinct from traditional and GenAI approaches that focus on specific tasks and content creations, long-thinking AI builds upon these fundamentals to tackle more intricate and complex problems.
While long-thinking AI holds a lot of power to tackle complex problems and challenges, its successful venture will depend upon its responsible deployment that benefits the large audience.