AI to Predict Wildfire And Reduce Damage
Wildfires are out-of-control fires that burn through forests, grasslands, peatlands, and pasturelands. AI to predict wildfire and reduce damage is the ultimate solution to decrease the risk of destruction, life and property loss. For it, extraordinary system training ways are necessary.
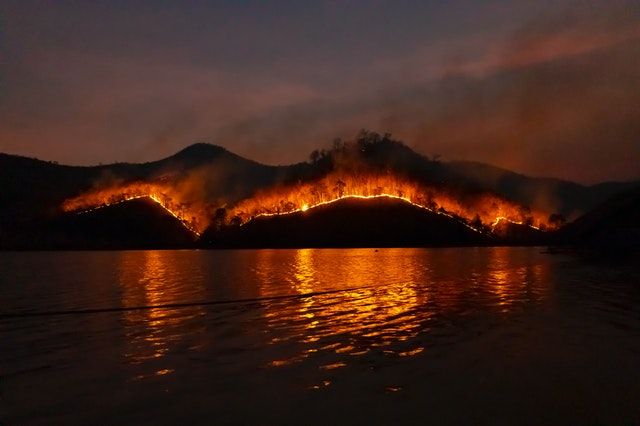
WILDLAND FIRES
Wildfires are uncontrolled fires spreading across forests, grasslands, peatlands, and pasturelands. This year was reported as another severe one regarding wildfires. These have significant impacts on the economy, wildlife, and humans. Globally, wildfires drive greenhouse gas emissions and are responsible for 8% of 3.3 million annual premature deaths due to poor air quality. According to the US Drought Monitor, about 90% of Western land experienced moderate to severe drought by August 31. About 6.6 million acres were burned in 2020 on November 26 compared with 8.9 million during the same period. In 2018, California’s total wildfire costs were calculated to be $400 billion annually; firefighting costs, property damage, economic losses (direct and indirect), and recovery expenditures are included.
Intensive AI models and excellent training are required to predict wildfire to reduce damage chances. What would cause the greatest reduction of wildfires? Let us discuss some ways.
1. PREDICTIVE MODELS
1.1. AI and Cameras in Remote Areas
One of the significant efforts to predict forest fire is the implementation of AI and cameras in various remote regions. The artificial intelligence used in them should be efficient enough in recognizing a morning fog and smoke plume rightly. Eight hundred cameras are distributed over Nevada, California, and Oregon. Even people who do not know science can remotely watch forest fires in real time. It helps fire managers to start making tactical strategies based on the situation and risk of wildfire before the firefighters reach the area.
Moreover, wildfire dealers call military fire drones randomly from the Air Force or National Guard to fly over the forests at night to see hot spots and boundaries. These drones use heat imaging technology for mapping hot areas. For viewing smoke and ash, these devices use satellite imagery. Wildfire policy expert and professor at Pomona College in California, Mr. Char Miller, said that firefighters' job is to manage the fire via these wild fire tools with a high degree of accuracy.
1.2. Forest Dryness Sensors from Space
Current vegetation methods to measure dryness demand much care. First, the team members find different branches in a specified area and place them in the oven to remove moisture content. Vegetation is weighed before and after oven drying. The difference between the two weights refers to the moisture content in branches. It is known as live fuel moisture content calculation that helps analyze the risk of wildfire in a given place. The data and information obtained are stored in the National Fuel Moisture Database by the US Forest Service. The database already has thousands of measurement data from the last half-century.
How can live fuel moisture be determined?
LFMC (live fuel moisture content) involves determining moisture content in fuels, including trees and shrubs, relative to their biomass when dried. Lower LFMC is directly related to the high risk of wildfires as fuels are dry and vice-versa.
However, different strategies are used to determine the dryness of different plant species as they respond to droughts uniquely. Some plants store water by closing their stomata, while others may not. That’s why it is challenging to map which species grow where and how. Wildfire models are not trained on these effects as they may predict wrongly. US Forest Service collects thousands of forest dryness measurements used to train a neural network with satellite data for forest dryness estimation in the western US.
The team specialized in hydrology, environmental engineering, and remote sensing developed a deep learning model to check the critical live fuel moisture content level all around the state from Colorado, Texas, Montana, and Wyoming to the Pacific coast. Researchers cannot see the amount of water inside all plants, making it challenging to determine forest dryness. Further, the geometry of vegetation canopies is also tricky to find. The scientists estimate forest dryness of only 5 to 17 vegetative plants. The detailed model preparation and its applicability are described in this paper. However, the model requires further testing can find forest dryness levels by uncovering pixel by pixel showing the areas at greater risk of catching fire. According to the lead author, Krishna Rao, the model is prepared using a recurrent neural network (an AI system that recognizes patterns in a large amount of data after learning). An ecohydrologist at Stanford University said that they worked on a newer set of satellites using longer wavelengths which help in:
- Seeing much deeper in vegetation canopies of forests.
- It directly shows LFMC.
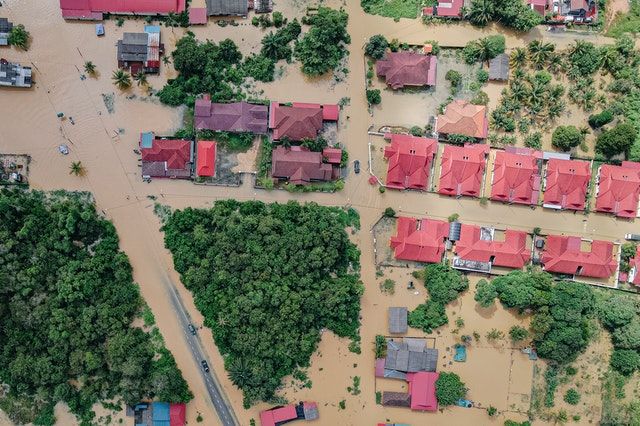
The team trained their model using three years of data related to the 239 sites in the western US. Then they looked at their developed system moisture predictive level across typical land covers like grasslands, shrublands, deciduous forests, and others. Their predictions were compared with the National Fuel Moisture Database to check its accuracy. The best results were shown for shrublands existing in the West and covering 45% of the land region. These regions proliferate and exhibit a significant risk of wildfire. That is why a better understanding of these ecosystems is the right way to understand fires and reduce risk.
1.3. Deep Learning Model to Map Fuel Moisture Level
The Fion fire detection system eliminates the need for offsite repeating visits with accuracy. It is an intelligent wildfire startup based in the San Francisco Bay Area. The tech startup uses deep learning models to help consumers better understand the problem, save lives, and protect properties. The technology provides four robust solutions.
FIRE PREDICTION: They predict most at-risk areas for wildfires and notify the authorities of locations with control solutions.
FIRE DETECTION: Fion company detects fires in real time to avoid the chances of enormous loss.
SPREAD PREDICTION: Their system uses 12 data sources to alert the clients about the exact fire spreading locations via satellite imagery, vegetation indices, wind vectors, and topography.
DESTRUCTION ESTIMATION: The only company worldwide that estimates destruction accurately is Fion. They calculate the financial damage caused by the fire in a specific area using APIs.
Z-FIRE is a data-driven model developed by a cooperation of American property insurance with Zesty.ai, a climate risk analytics startup. Their purpose is to introduce Z-FIRE into its homeowner product line to write policies confidently. This AI model trains over fourteen-hundred wildfire events with missing data from more than twenty years. The model analyzes historical climate data and topography. Further, it analyzes building materials and vegetation around in multiple spaces. Then it generates a predictive risk score and helps homeowners find mitigation points before any bad event happens. Z-FIRE provides solutions to insurers on how likely a home is exposed to wildfire and what damages it can cause.
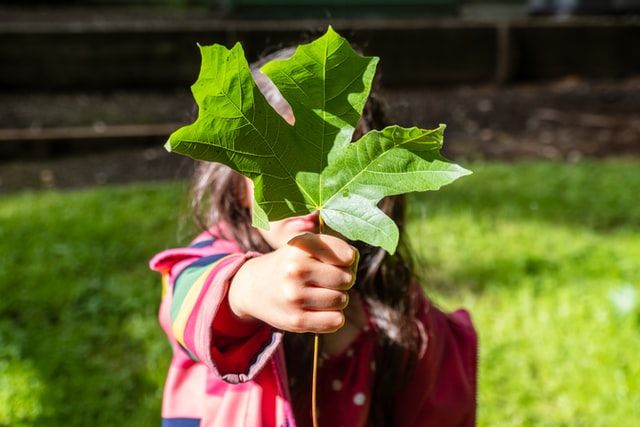
1.4. Alchera’s Visual Anomaly Detection System
A wide array of information is required to predict wildfires with AI, including satellite images, wind forecasts, and drone surveillance. Then the intelligent system’s provided data is used to mitigate fire risks by taking related steps. Sonoma County awarded Alchera Inc. of Seoul, South Korea, $300,000 to develop a fire detection system based on machine learning technology that can be added to the Wildfire Alert Network of the county. The visual anomaly detection system spots forest fires by scanning standard video. The company collected millions of video images by remote cameras and used them to train their fire-detection algorithm. Its engineers claim that their fire system is the first commercial software providing real-time services. Sahota, chief officer of innovation at the University of California, said that their team is working on prediction technology better than just automation. Alchera and ALERTwildfire are paired together to provide "image recognition AI" that sorts images exceptionally higher than humans.
2. DAMAGE CURE
2.1. AI Algorithms to Fight with Future Wildfire
A NASA computer engineer, James MacKinnon, said that an algorithm could be run on satellites that help image processing in a few minutes. He scaled down the work done by supercomputers in a neural network. He took a year to train the system with 98% accuracy at recognizing fires. He says the fires appear like a sore thumb via his trained model. AI-based systems in the future connected with satellites will provide more consistent contact with Earth. It, in this way, will send real-time alerts to take emergency actions if needed by responders on the ground.
One way to stop wildfires is to highlight the vulnerable areas to new fires and prevent them before ignition. California Forest Observatory (CFO) is a joint project started by Salo Sciences and Planet; CFO maps various drivers for wildfires, including weather patterns, vegetation lives fuel content, topography, and infrastructure by using real-time images from Western sentinel (1 and 2), and Planet Labs satellites. They strive to improve community and forest resilience in high fire-risk areas to provide agencies like CALfire with accurate and real-time details about wildfire threats all over the state.
2.2. Spotting Fires Quickly- Descartes Lab
One common issue with wildfires is that they are spotted too late and spread long. Descartes Lab, a Santa Fe-based startup, strives to eliminate this delay in fire prediction by training its AI system to spot budding blazes. The AI software is connected with two US weather satellites and scores images every ten minutes. It finds hotspots like changes in thermal infrared data and alerts the fireteam before any unusual event happens. Descartes lab researchers are now testing the detection efficiency of their system by sending alerts to the department’s heads. Since it was launched two years ago, the initial results were promising; the system spotted over 6000 fires (including a small fire of ten acres) in less than nine minutes. Several agencies also installed ALERTwildfire cameras that is a leading system in California that uses artificial intelligence to detect firs. It is a tower-mounted with twenty-one pan-tilt-zoom fire cameras linked with AI software to survey the county's surfaces for smokes and flames. After every ten seconds, the cameras send images to AI to compare coming pictures with historical images of the exact location for detection if any risk persists. In an unusual case, the emergency crews are notified in real time to take immediate action. The company comes with more than 800 cameras across eight states, but only the Sonoma County alert system has introduced an intelligent system.
2.3. Computing Blazing Path (WIFIRE Lab)
The wildness of wildfires makes them even more dangerous than they are actually. The fires just need ignition and then spread at varying speeds and change their direction to any side, destroying everything. Now fire agencies are using the FireMap tool based on artificial intelligence developed by WIFIRE Lab at the University of California. It's efficient at creating a predictive map in minutes regarding the expected tragedy of fire spread. The system uses deep learning techniques to provide real-time data related to vegetation dryness, topography, and weather by collecting information from satellites, sensors, and cameras. The principal investigator at WIFIRE Lab described that the team fed all information gathered into the models that then predict fire, its spreading rate, and direction of spread up to six hours.
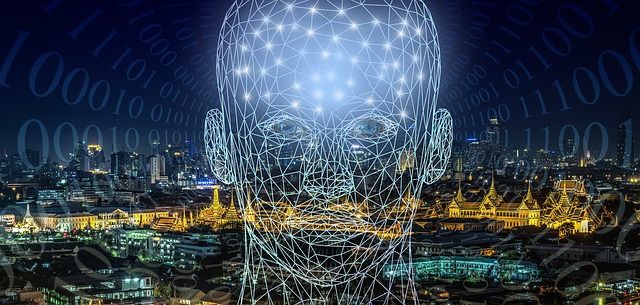
The WIFIRE system uses wildfire tools including a fixed wireless network that connects the supercomputer center at San Diego with other remote weather stations and high-speed fiber optics for sending information in less than a second. The system provides high-resolution weather data that help the WIFIRE model predict the way of fire spreads.
Briefly, it offers information that helps fire departments deploy their limited sources efficiently. Wildfires are becoming more common and destructive with changing climate; firefighters need better techniques to know about them before any life or property loss. National Science Foundation funded the WIFIRE project $2.6 million, but its scheduled end date has been reached recently. About $200000 is required per year by Altintas to maintain WIFIRE. Prominent associations pledged to contribute $25000 per year and hope to get the remaining amount from the government to keep the system operational.
2.4. Fire Fighting Drones
What is the best way to fight a fire? Drones are promising technology from portable quadcopters to fixed wings as they have advantages over conventional firefighting technology. Helicopters and airplanes used to survey wildfires cannot fly in dark and smoky conditions. Similarly, flying over raging also puts pilots’ life in danger. Drones also involve finding critical live fuel moisture content in forests. According to the US Forest Service, about a quarter of all firefighters' (wildland) deaths occurred due to aviation. The fire got uncontrolled last summer in West US, and about 24 remotely controlled devices provide real-time data and high-resolution footage of fired forest. All were equipped with thermal imaging cameras. In this way, the drones helped to get the critical information for making decisions at the right time that won't be possible in any other way. Kelly Boyd, a drone specialist, brought an ignis funnel-shaped system developed by Drone Amplified that can drop 450 small balls in about four minutes.
Dragon egg fire fighting drones are ping-pong spheres like balls filled with two chemicals that react after hitting the ground. They start small fires to disturb the path of an approaching fire to stop its fuel from spreading. According to Boyd, airborne firebombing is an excellent and versatile way to stop wildfires. Further, he is expecting that in coming years, drones of future firefighting technology will stay in the fires for days and send continuous video streams with teams to help drench flames where human-controlled vehicles are not expected to move.
2.5. New Silicon Valley Lab
Silicon Valley-based lab for AI development will include an efficient model to predict and resist wildfires in real time. CMM (Cognitive Mission Manager) system is an end-to-end AI-driven platform that joins real time fire sensor data with other sources, including topography, fuel vegetation, and wind, to predict the fire expanding probability. The system offers recommendations to the relevant teams for improving the efficiency of wildfire suppression. The lab is open for industries and users to gather talent and resources for CMM design improvement and prototypes development in NVIDIA Omniverse. Lockheed Martin uses NVIDIA Omniverse Enterprise for fire visualization and predicts expansion of wildfire speed. Moreover, NVIDIA DGX is used to crunch the data and train AI models accordingly. Shashi Bhushan, principal AI at Lockheed Martin, said that the collaboration of NVIDIA and Lockheed helps teams respond quickly to wildfire while reducing life risks to residents and fire crews.
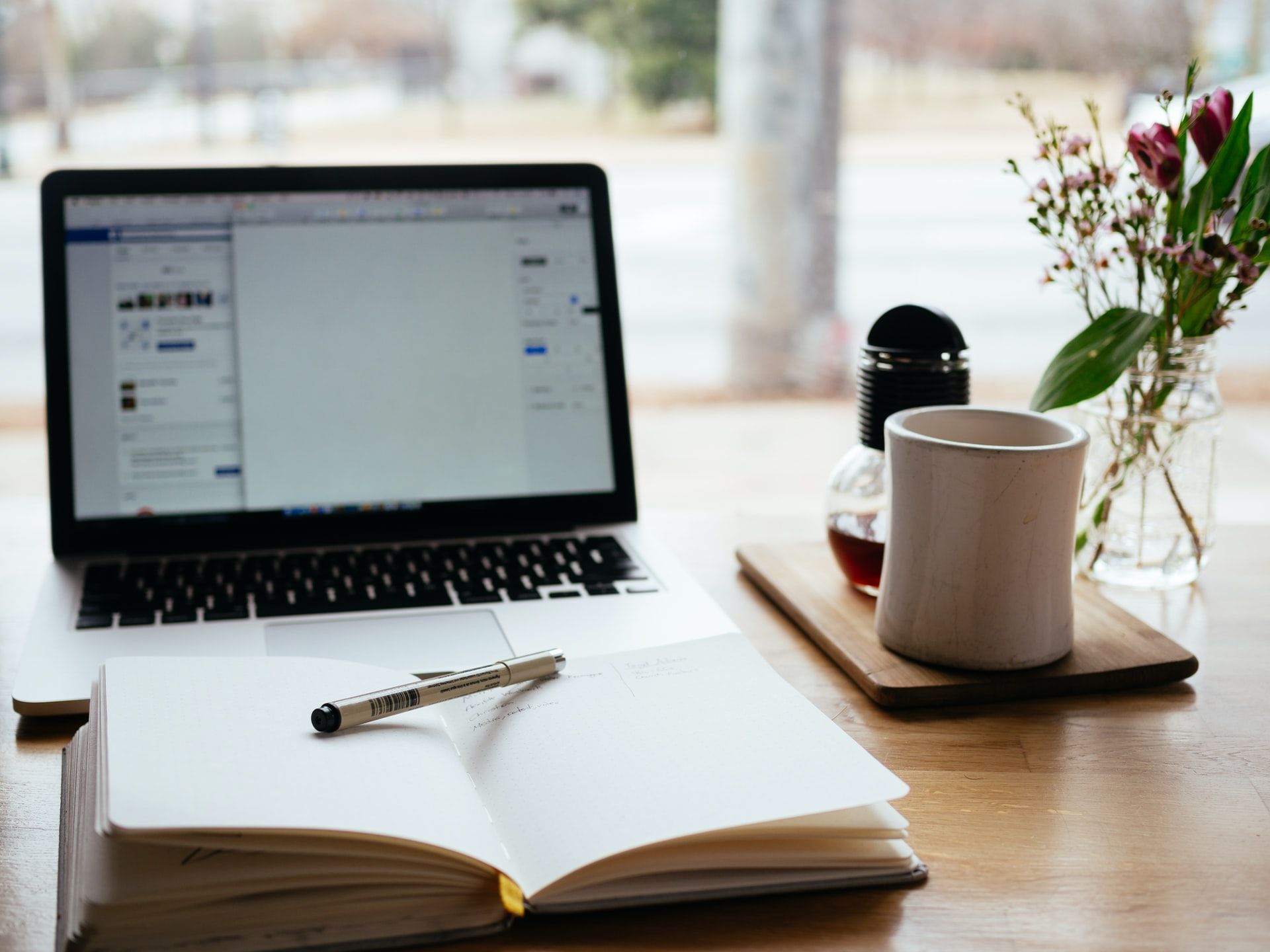
2.6. Charcoal Particles Involved in Predictive Modeling
Climate change is a significant driver of increased wildfire risk. According to predictive climate models, warm and dry conditions promote fire and will be more intensive than this century in the future. Climate change made the US dryer and hotter in the last 30 years, and scientists have warned the government that the weather will be hotter as the world warms.
The researchers from Brown University studied sediments core from Swamp Lake bottom to check the effect of long term environmental changes. Heavy fires burned the northern area around Yosemite National Park, and smoke plumes fell into the lake, sank to the bottom, and became sediment over time. They tracked change in charcoal content as going more profound in the lake and recorded how much data had burned over the last 1400 years to 2007.
The team tracked local and regional fire activity by determining the size of charcoal particles. Small-sized particles can move farther in air recorded regional fire in about a 90-mile lake radius. In contrast, bigger-sized particles cannot move so far and recorded fire activity within 15-miles. The team correlated climate change with fire risk by synchronizing collected charcoal data with climate records based on temperature and rainfall calculation.