Building Trustworthy AI through Explainable AI (XAI)
Explainable AI (XAI) sheds light on how AI makes decisions, bridging the gap between complex models and human understanding. XAI reveals which factors most influence AI's choices. This transparency builds trust in AI, allowing experts to improve its performance and unlock its full potential.
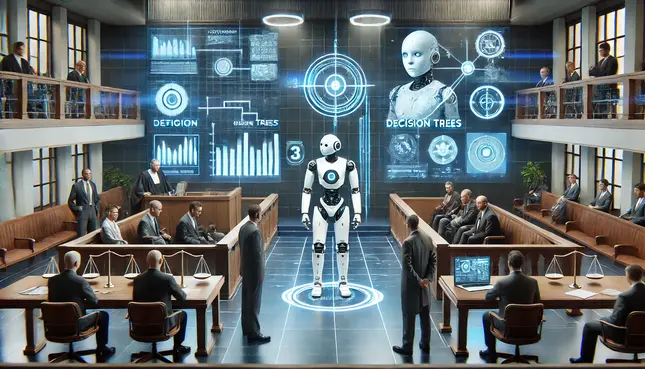
Imagine super-smart machines making choices on their own, like self-driving cars without a steering wheel or assistants that understand you better than your best friend! That's the power of Artificial Intelligence (AI). But here's the catch: how do we know how these machines actually reach their decisions? It's like a magic box, the answer pops out, but nobody knows the steps it took to get there. This is where Explainable AI (XAI) comes in.
What is Explainable AI (XAI)?
XAI acts like a detective, figuring out how these complex AI models actually make their choices. By understanding these steps, we can be sure the AI is working correctly and isn't making any unfair decisions. Plus, it helps us improve these machines to be even smarter in the future!
Explainable AI (XAI) refers to techniques that help us understand the reason of which a decision has been made. It aims to shed light on the "black box" of AI, where complex models often produce decisions that are difficult to interpret. Imagine using a GPS system that tells you to take a route without explaining why it chose that path. Won’t you like it if it provided reasons, like traffic conditions or road closures? This analogy captures the essence of XAI.
The importance of explainability in AI systems cannot be overstated. It is crucial for building trust, ensuring accountability, and enhancing the usability of AI applications. Unlike traditional "black box" models, XAI strives to provide transparency and interpretability.
Explainable AI Global Market Forecast
According to Markets and Markets, the explainable AI (XAI) market is showing rapid growth, compared to its previous value increasing from $5.1 billion in 2022 to $6.2 billion in 2023, and it is expected to reach $16.2 billion by 2028. This growth represents a compound annual growth rate (CAGR) of 20.9% over the forecast period.
Why do we need Explainable AI (XAI)
We need Explainable AI (XAI) for several key reasons. Here's a breakdown:
- Trust and Reliability: Many AI applications operate in critical domains like healthcare or finance. Without understanding how these models reach their decisions, scientists struggle to assess their trustworthiness. If a doctor can't explain why an AI recommends a specific treatment, it can be difficult to confidently rely on that recommendation.
- Bias Detection and Mitigation: AI models may inherit biases from the data they're trained on. XAI techniques allow scientists to identify these biases within the model's decision-making process. Once these biases are revealed, they can be addressed through adjustments to the training data or the model itself, promoting fairer AI development.
- Debugging and Improvement: When AI models make mistakes, XAI helps scientists identify the root cause of the error. If we understand why the model made a specific wrong decision, scientists can refine the model's logic and improve its overall performance.
- Model Understanding and Knowledge Discovery: XAI sheds light on the complex inner workings of AI models. This can lead to new scientific discoveries about the relationships between variables within the data itself. Scientists can use these insights to develop more effective models and gain a deeper understanding of the systems under observation.
Explainable AI vs Interpretable AI vs Transparent AI
In the world of XAI, three terms get thrown around a lot: explainable AI, interpretable AI, and transparent AI. While they all aim to make AI models less like mysterious black boxes, there are subtle differences between them.
Let’s differentiate between three terms using an analogy. Imagine you're a doctor trying to diagnose a patient's illness.
Transparent AI
This would be like having a completely see-through body for the patient. You could directly observe all the organs and systems working together, making the diagnosis straightforward. However, this level of transparency might not always be feasible or desirable in complex AI models.
Interpretable AI
This is like having a simple medical chart that clearly outlines the patient's symptoms and medical history. Based on established medical knowledge and rules, you can arrive at a diagnosis using a well-understood process. Interpretable models are easy to understand but may not capture the full complexity of a real-world situation.
Explainable AI
This is like having a skilled doctor who can explain the reasoning behind the diagnosis. They might use test results, medical knowledge, and their experience to explain how they reached their conclusion, even if the inner workings of the human body remain a complex mystery. Explainable AI focuses on making the decision process understandable, even for intricate models.
Key Terms in Explainable AI (XAI)
- Local vs. Global Explanations: Think of these as a detailed medical report vs. a patient's overall health history. Local explanations delve deep into a specific instance, like why a particular patient was diagnosed with a specific condition. They analyze factors like blood tests, X-rays, and symptoms for that individual case. Global explanations, on the other hand, provide a broader picture. They look at trends across a population, examining how factors like age, genetics, and lifestyle might influence the overall prevalence of certain diseases. Similarly, in AI, global explanations might analyze how different features commonly influence the model's overall behavior.
- Feature Importance: This concept is like identifying the most critical medical tests for diagnosis. Just as some blood tests might be more indicative of a specific illness than others, feature importance helps us understand which input features in an AI model have the most significant influence on the final output.
- Counterfactual Explanations: These are like exploring "what-if" scenarios in medicine. Imagine asking the doctor, "If the patient had a slightly higher white blood cell count, would the diagnosis change?" Counterfactual explanations in AI work similarly. They analyze how a model's prediction might change if we tweak a specific input data point. This can be helpful for understanding the model's reasoning and identifying potential biases. For instance, you could ask an AI why it rejected a loan application, and the AI might explain what income level would have secured approval based on the model's decision logic.
By using these XAI tools, we can gain valuable insights into the decision-making process of AI models, fostering trust, fairness, and ultimately, better AI development.
Explainable AI (XAI) isn't just theoretical, it's making a real impact in various fields. Here's a glimpse into how XAI is transforming how we interact with AI systems:
1. Ensuring Fairness in Loan Approvals:
- The Challenge: Traditional loan approval models can perpetuate biases based on historical data. For instance, if a certain demographic group was historically denied loans more often, the model might continue this bias.
- XAI in Action: By using XAI techniques like feature importance, we can identify which factors have the most significant influence on loan decisions. This helps lenders identify and mitigate potential biases, ensuring fairer loan approval processes.
2. Improving Medical Diagnoses:
- The Challenge: AI models are increasingly used to assist doctors in diagnosing diseases. However, a lack of transparency can make it difficult for doctors to understand the AI's reasoning behind a diagnosis.
- XAI in Action: XAI techniques like local explanations can help doctors understand why a specific diagnosis was suggested by the AI model. This allows doctors to leverage the power of AI while maintaining their own medical expertise for a more comprehensive diagnosis.
3. Building Trustworthy Self-Driving Cars:
- The Challenge: For self-driving cars to gain public trust, people need to understand how they make decisions, especially in critical situations.
- XAI in Action: XAI can be used to explain a self-driving car's decision to change lanes or brake suddenly. This transparency can help passengers understand the car's reasoning and build trust in its autonomous capabilities.
4. Simplifying Fraud Detection:
- The Challenge: Financial institutions often use complex AI models to detect fraudulent transactions. However, without understanding how these models identify fraud, it's difficult to determine if a flagged transaction is a true positive or a false alarm.
- XAI in Action: XAI techniques like counterfactual explanations can help analysts understand what specific factors triggered the fraud alert. This allows them to investigate suspicious activity more effectively and reduce the likelihood of wrongly flagging legitimate transactions.
5. Enhancing Explainability in Customer Service Chatbots:
- The Challenge: Chatbots are often used for customer service, but their responses can sometimes feel dismissive or lack context.
- XAI in Action: By incorporating XAI principles, chatbots can explain their reasoning behind suggested solutions or answers. This transparency can improve user experience and build trust in the chatbot's ability to assist customers effectively.
Subcategories of Explainable AI Techniques
We've explored the need for XAI and its real-world applications. Now, let's delve deeper into the toolkit itself! XAI techniques can be broadly categorized into two main subcategories:
- 1. Model-Agnostic Techniques: These versatile tools can be applied to explain a variety of AI models, regardless of their internal workings. Think of them as universal wrenches that can fit many different nuts and bolts.
- 2. Model-Specific Techniques: These techniques are designed to exploit the specific structure and properties of a particular type of AI model. Imagine having a specialized screwdriver set that works best for specific types of screws.
Here's a breakdown of some key techniques within each subcategory:
Model-Agnostic Techniques
- LIME Explainable AI (Local Interpretable Model-Agnostic Explanations): Imagine a complex AI model as a black box. LIME works by creating a simpler, easier-to-understand model around a specific data point. This local model helps explain why a particular prediction was made for that data point. (Think of creating a local map to explain how someone navigated a maze, even if you don't have the entire maze blueprint.)
- SHAP Explainable AI (SHapley Additive exPlanations): Inspired by fair allocation methods, SHAP distributes the credit for a prediction among all the input features in an AI model. This helps us understand how each feature contributes to the final outcome, similar to how each player in a team contributes to the overall win.
- Feature Importance Techniques: These techniques, like permutation importance or sensitivity analysis, rank the features based on how much the model's output changes when a particular feature is shuffled or modified. This gives us a quick idea of which features hold the most sway over the model's decisions.
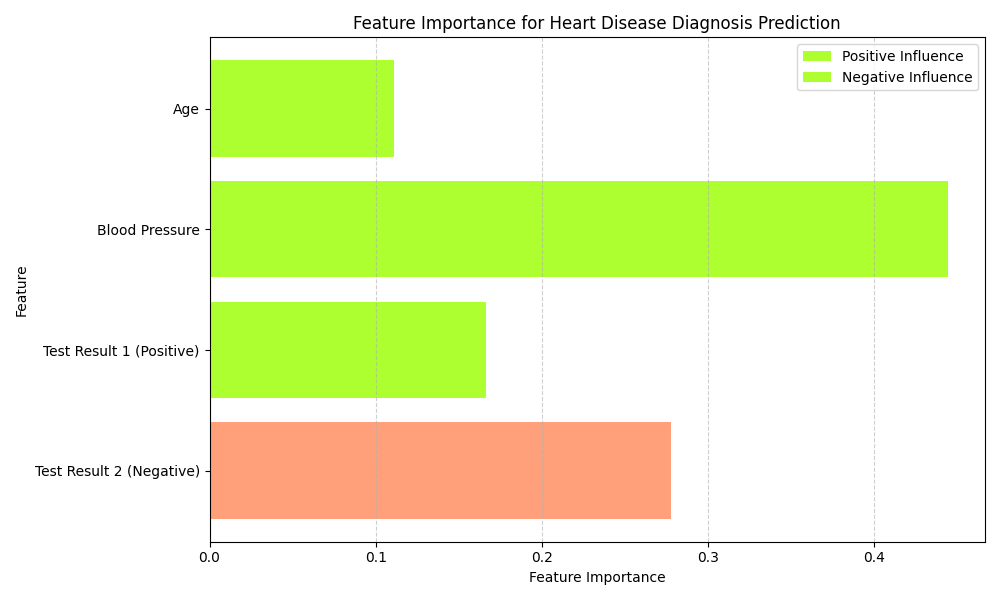
- Anchors: This technique leverages simpler models to explain the predictions of complex models. Imagine having a simpler friend explain the decisions of a more complex chess master. By comparing the reasoning of both models, you can gain insights into the complex model's thought process.
Model-Specific Techniques
- Explainable Decision Trees and Rule-Based Models: These models are inherently interpretable by design. The logic behind each decision is explicitly encoded in the tree structure or the set of rules, making them easy to understand for humans. (Think of a flow chart where each step and decision point is clear.)
- Saliency Maps for Deep Learning Models: Deep learning models are powerful but often opaque. Saliency maps create a visual heatmap highlighting the parts of the input data that most influenced the model's decision. Imagine a blurry image where the most important areas are brought into focus to reveal what caught the model's eye.
- Counterfactual Explanations: This technique is particularly useful for complex models. It allows you to explore how a model's prediction would change if you slightly modified the input data. For instance, in a loan approval scenario, you could see how a small increase in income might affect the outcome. This can help identify potential biases and understand the model's reasoning process.
Challenges and Considerations in XAI
Trade-off Between Accuracy and Explainability
One of the main challenges in XAI is balancing accuracy and explainability. Complex models like deep neural networks are highly accurate but often lack interpretability. Simpler models, while more understandable, may not achieve the same level of performance. Finding a middle ground where models are both accurate and explainable is a key research area.
Evaluating Explainability
Measuring the effectiveness of explanations is another challenge. Various metrics and frameworks are being developed to assess how well explanations help users understand model decisions. These include fidelity (how accurately the explanation represents the model), completeness (how much of the decision process is explained), and interpretability (how easily the explanation can be understood).
Human Factors in Explainability
Tailoring explanations to different audiences is crucial. Domain experts and laypeople have different levels of expertise and require different types of explanations. For example, a medical professional might need detailed explanations of an AI diagnosis, while a patient might only need a simplified version. Understanding and addressing these human factors is essential for effective XAI.
Bias in Explainable Models
Bias in AI systems can be perpetuated or even amplified by XAI tools. It's important to ensure that the explanations provided do not reinforce existing biases. Researchers are working on methods to identify and mitigate bias in explainable models, ensuring fairness and equity in AI systems.
Explainable AI (XAI) is rapidly evolving, with exciting developments in libraries, research areas, and its future potential. Here's a look at some key advancements:
Rise of Explainable AI Libraries and Toolkits
Developing explainable models is becoming easier thanks to dedicated libraries and toolkits. Here are two prominent examples:
- TensorFlow Explainable AI (TF-XAI): This open-source toolkit from Google offers various tools for developers to create interpretable models and generate explanations for their predictions. It supports a variety of explainability techniques, making it a versatile solution.
- SHAP (SHapley Additive exPlanations): While not strictly a library, SHAPley Explainable AI is a powerful framework for interpreting any machine learning model. It uses game theory concepts to distribute the credit for a prediction among all the input features, providing insights into individual feature importance.
Emerging Research Areas
XAI research is pushing boundaries in several areas:
- Explainable Reinforcement Learning: Reinforcement learning involves training AI systems to make decisions through trial and error. Traditionally, these decisions can be opaque. Explainable reinforcement learning highlights the system's decision-making process, making it easier to understand and potentially debug its behavior.
- Explainable Neural Networks: Deep learning models, especially complex neural networks, are often seen as black boxes. Explainable neural networks research focuses on incorporating explainability principles directly into the architecture of these models. This can lead to inherently more interpretable deep learning systems.
The Future of Explainable AI
The future of XAI looks promising, with a focus on:
- Standardization and Regulation: As AI becomes more integrated into various industries, ensuring explainability and transparency will be crucial. However, establishing standardized metrics and regulations for XAI can be challenging due to the diversity of AI models and applications.
- Social Good Applications: XAI has the potential to promote responsible AI development and address ethical concerns. By making AI models more transparent, we can identify and mitigate potential biases, fostering trust and fairness in AI systems. Additionally, XAI can be used for social good initiatives, such as helping explain complex medical diagnoses or improving decision-making in public policy.
Companies working on Explainable AI
1. Pioneering Research: DARPA's XAI Program
Imagine the US Department of Defense funding research to make AI decisions clearer! DARPA (Defense Advanced Research Projects Agency) has been a leader in XAI research since 2016. Their XAI program aims to develop explainable models for various applications, from military simulations to national security. This pioneering work is paving the way for a future where even complex AI systems can be understood and trusted.
2. Industry-Specific Solutions: IBM Explainable AI
Big Blue isn't sitting this one out! IBM goes beyond general Explainable AI (XAI) solutions and offers an industry-specific approach through WatsonX.Governance. This comprehensive suite empowers businesses like banks and healthcare providers to not only understand how AI models reach decisions, but also to govern and manage those models responsibly.
3. Democratizing Explainability: Google's AI Explainability Toolkit
Google offers a comprehensive suite of Explainable AI (XAI) tools. For developers working on Google Cloud Platform (GCP), Vertex Explainable AI provides functionalities like feature attribution and the What-If Tool to understand model behavior. Additionally, the open-source Captum Toolkit empowers developers beyond GCP with various explainability methods for different machine learning models.
Open-Source Tools and Libraries for XAI
- LIME (Local Interpretable Model-Agnostic Explanations): Imagine needing to explain a complex magic trick. LIME acts like a friendly assistant, simplifying even the most mysterious AI models. It creates easy-to-understand explanations for specific predictions, helping you grasp how the model arrived at its decision for a particular data point.
- SHAP (SHapley Additive exPlanations): Ever wondered how much credit each player takes in a winning team? SHAP brings that concept to the world of AI. It uses game theory to distribute the credit for a prediction among all the input features in an AI model. This helps you understand which factors have the biggest influence on the final outcome, making the model's reasoning more transparent.
- ELI5 (Explain Like I'm 5): This library lives up to its name! It aims to explain complex AI models in a way that even a curious five-year-old could understand. ELI5 uses techniques like text simplification and analogy generation to break down technical concepts into plain English. While not as in-depth as other XAI tools, it's a great starting point for beginners or anyone who wants a high-level understanding of how an AI model works.
Conclusion
Explainable AI is a critical area of research and development that aims to make AI systems more transparent, understandable, and trustworthy. By providing insights into how AI models make decisions, XAI helps build trust and accountability in AI applications. As we move forward, the potential of XAI to impact various fields is immense, and further research and development are essential.