Artificial intelligence, Enterprise AI, Data Science, Big Data, Robotic Process Automation, Augmented Reality, Digital Transformation, Fintech and many other buzzwords are becoming talk of the town these days aiming to automate, optimize and improve business processes and customer experience. Companies are rapidly shifting towards AI-driven technologies to transform traditional business workflows and achieve business goals. The final results may meet expectation, but there is a huge risk of failure attached to it that is less thought of.
AI and Data Science technologies are much improved and advanced now compare to 10 years ago but there is lot more to improve when it comes to meeting end-user expectations and real-life implementation of an Enterprise AI project. AI operations and processes is one factor but there are many other reasons that lead to failure of data science projects. These include:
- Absence of comprehension about AI tools and methodology.
- Lack of investment in employees who know data very well.
- Not opting the right tool.
- Poor Data Quality.
- Bad Strategy from top management.
In the end of the article we have briefly discussed the reasons - why AI projects fail?
AI is an evolving technology. It is imperative to do continuous in-depth research on a particular topic. Many AI projects fail before time in filling the conventional gaps. Last year, many big sites predicted that major data science projects would face failure in the future. According to a report, 87% of ongoing projects will fail in delivering the desired results. According to the expert's report, AI growth will result in moral issues of business users and consumers. In 2017, 73% of developers decided to end working with advanced technology in 2018, and some others didn’t plan to use AI in future. Even space startups fail this year due to several reasons including; inexperience workforce, lack of expertise, ideal expectations, lack of funding, and other technical & non-technical issues.
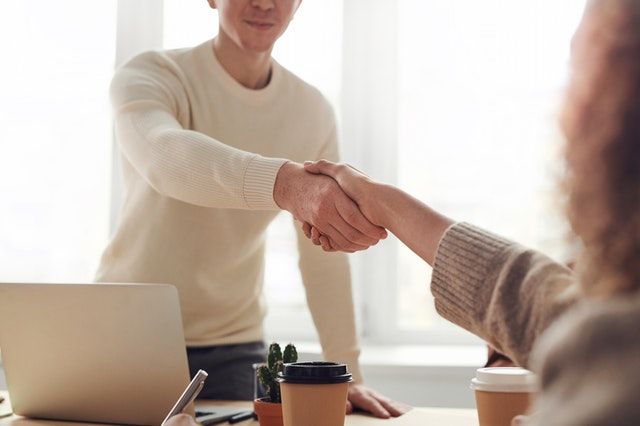
Here is the list of 5 biggest failures of AI in the past few years that failed to fulfill investor’s expectations.
Failure 1: IBM’s Watson for Oncology Project Cancelled After Spending $62 Million
Reason: IBM joined with the University of Texas MD Anderson Cancer Center for the development of an advanced Oncology Expert Advisor system. Its mission was to cure cancer patients. The press highlighted the first line as:
"MD Anderson is using the IBM Watson cognitive computing system for its mission to eradicate cancer. Its primary aim is to uncover valuable information for the cancer centre's rich patient and research database."
In July 2018, StatNews studied IBM’s internal documentation for this project; they found it too dangerous for treating cancer patients. StatNews blamed IBM’s engineers for this careless attitude in recommending unsafe treatment. They trained Watson on relatively smaller dataset and ignored other significant features related to cancer patients. Watson discovered reasonably easily how to review clinical trial papers and assess the underlying findings. But teaching Watson to read the papers the way a doctor might, proved impossible. The data that doctors extricate from an article, that they utilize to alter their care, may not be the major point of the think about in this project. The approach of Watson is focused on numbers, compile statistics and outcomes. Doctors don't function that way though. When applied in real world, IBM found that its ground-breaking innovation is no counterpart for the untidy truth of the present medical care framework. Also, in attempting to apply Watson to cancer treatment, probably the greatest test, IBM experienced a central befuddle between the manner in which machines learn and the way physicians work. Furthermore, the software also recommended doctors to treat cancer patients with bleeding drugs; that will eventually increase bleeding and make the condition worsen. A doctor at Jupiter Hospital in Florida told IBM representatives according to the study:
“We bought it for marketing and with hopes that you would achieve the vision. We can’t use it in many cases."
In February 2017, the University of Texas Auditors reported that MD Anderson spent $62 Million without getting the achievement.
Failure 2: Apple’s Face ID Fail
Reason: The well-known Apple Brand developed a facial recognition ID system over the fingerprint sensor as chief passcode. The technology failed here in providing extra security layer as a plastic mask succeeded in making it fool. The given Apple device is not right for people who are significantly concerned with their privacy issues.
It was Apple iPhone X with generally positive reviews. The company said that the device consisted of a front-facing camera and machine learning (ML). Both components helped in creating the three-dimensional shape of its user's face. They introduced Artificial intelligence to detect cosmetic changes (user with make-up), pair of glasses on face, or wearing a scarf; they thought it would help in enhancing security, but the opposite scenario happened. Hackers were already claimed to defeat this technology by using 3D Printed Masks, and after its launching, they started making related attempts. Soon, Vietnam-based security company Bkav contended that they could successfully defeat Apple's Face Lock ID by joining 2D "eyes" with a 3D mask. The mask costs around $200, which is made up of stone powder and eyes were simple infrared (IR) printed images.
Apple first declared that its face ID would protect the device from fake masks by using anti-spoofing neural networks. However, Bkav’s work was insufficient to convince everyone. Wired wrote an article about Bkav’s announcement that discussed some doubts about their work by a researcher, Marc Rogers from Cloudflare, a security firm.
Failure 3: AI Robot Failed in Getting Admission at the University of Tokyo
Reason: The researchers tried to develop a robot Todai, to crack the entrance test for the University of Tokyo. Its one of the tasks that only humans can do with required efficiency but researchers thought they could train machines for this purpose. Unfortunately, the results were opposite to their expectations as AI was not smart enough in understanding the questions. It would be better to introduce a broad spectrum of related information in the robotic system; so, it can answer the questions rightly.
Respective members from the National Institute of Information gave their statement about Todai:
"It is not good at answering a type of question that requires the ability to grasp the meaning in a broad spectrum."
They have started working on the project in 2011, and it scored high marks in mock tests for getting admission in the University of Tokyo. But because of its inefficiency, they are eager to develop a better one in 2022. Those limitations inspired them to make it more reliable than its first version. The researchers from Japan will shift their focus on academic study skills that are required for a written response.
Failure 4: Facebook Struggling to Keep Hate Content Away
Reason: Facebook is one of the giant social media platforms that have already made significant amendments in their systems. But still, its efficient Artificial Intelligence system is unable in predicting hatred and illegal content. It seems to be a distant reality that advanced algorithms detect negative posts and content and don’t allow the user to upload it.
The company is spending more time on humans as they train machines according to human thinking strategy. Thus, it will help them in detecting problems for this delay and finding the solutions for it. Some of the many reasons that Facebook faces in introducing the desired system are:
- Lacking the right data for training AI algorithms.
- Development of right programs for detecting hate content.
Failure 5: Amazon’s Facial Recognition Software Falsely Recognized the U.S. Congresspeople
Reason: The American Civil Liberties Union showed in 2018 the failure of Amazon's AI facial identification system. According to their report:
“Nearly forty per cent of Rekognition’s false matches in our test were of people of colour; even though they make up only twenty per cent of Congress."
However, it was not the first time in which the system recognized someone falsely. The University of Toronto and MIT research specialists revealed that every facial identification system worked best for lighter-skinned faces. Furthermore, they found fault occurs in every one case out of three in recognizing darker-skinned ladies. The system is capable of responding and detecting faces with fifty per cent accuracy.
It's not only the fault of AI, but all the systems, organizations and above all, expert humans dealing with it are responsible. Law enforcing agencies are also working with various tools like Rekognition for precise identification of objects. Even Amazon's system is badly failed in delivering what's expected; Amazon is still selling Rekogition.
Why AI Fails in Delivering What’s Expected?
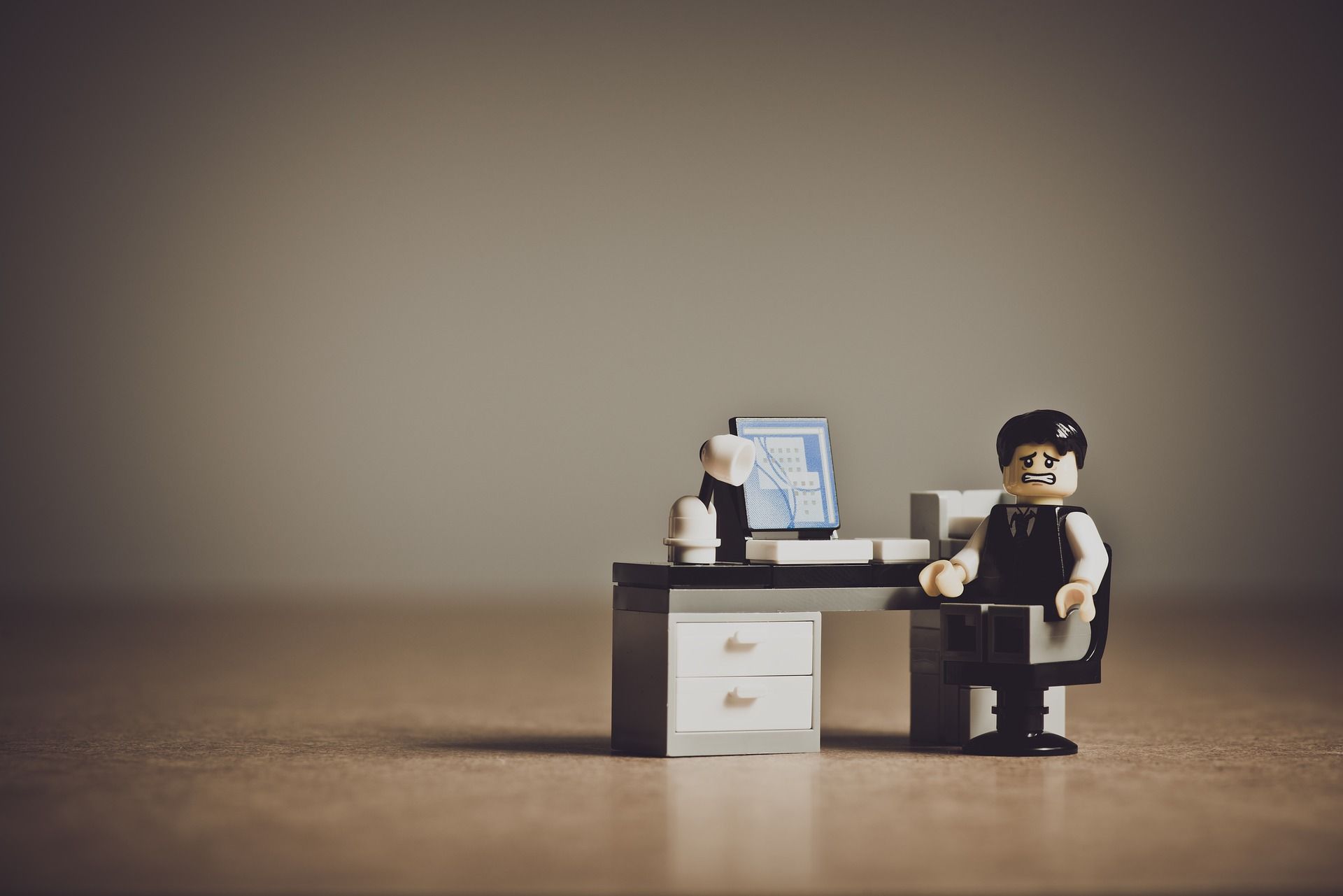
There might be several reasons, but the given are significant factors that must be considered for making the system accurate.
Insufficient Data
Data is the most critical factor in training Artificial Intelligence, according to it. Researchers use the right data to train statistical models with deep machine learning algorithms. Primarily, millions of data coding are necessary for proper building and working of an AI system. The data must follow the pattern of a real-world scenario without any bias; otherwise, it will lead to failure.
Bad Engineering
It's tough to spot a particular issue while detecting the reasons for failure in the AI system. However, faulty engineering leads to wrong neural network settings, even when the data is accurate. But the above examples discussed are about highly responsible companies; they can afford the best engineers.
Complex Area of its Application
It might be a reason that the system under consideration is highly complex and need data that is difficult to obtain. Sometimes, the results obtained should be highly accurate to develop a precise algorithm. For instance, the usage of AI techniques for the medical industry, law, and other complex industries will be complicated. It requires active human minds, efficient workforce, and enough information to develop an accurate system.
How to Remove Errors in Developing Excellent AI System?
For the development of a unique system, the researchers need clean, simple, and verified data to train machines according to it. In addition to data, choosing the right algorithm and testing it for different parameters is also the demand. Thus, it requires expert engineers to perform this exceptional task.
Point to Consider: All Failures are NOT Bad
Despite many incomplete AI promises which are irritating, it's essential to think that all failures are not wrong in real. The director of Cognitive Automation and Innovation at ISG, Wayne Butterfield, said:
“Finding how not to do something might be a success. It’s relevant in the world of AI and data; so, we need to be careful in broad-brushing failures.”
AI system has a minimal approach to replace humans altogether. It can help humans in performing daily repeated tasks but can't replace them in dealing with complex systems. Otherwise, it will lead to errors by AI. The best use of AI is to assist humans as a tool in performing daily tasks with high efficiency. You can't anticipate that AI should mirror the tasks and complexities of the human mind, yet you can anticipate that it should precisely predict things for you.
Cheers :)