Harnessing AI's Power: Transforming Historic Weather Data into Predictive Insights
AI analyzes extensive data to revolutionize weather forecasting in real-time and provide timely information to forecasters. AI models are trained on historical weather data for accurate predictions. AI can help address climate change by gathering and analyzing data, and hence, creating forecasts.
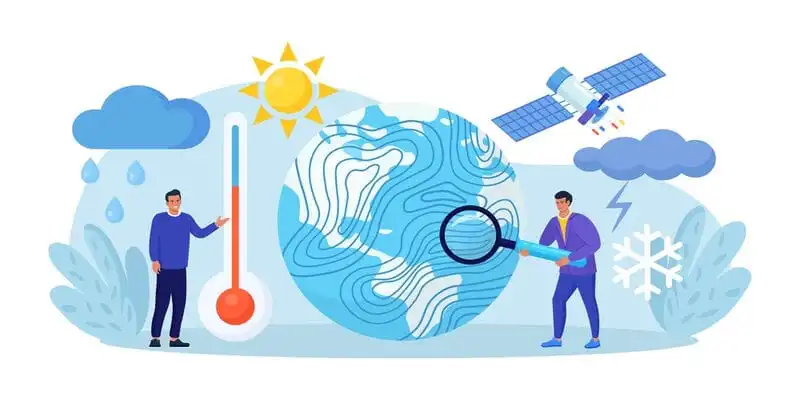
In a world where climate change and weather uncertainties increasingly impact our daily lives, the ability to accurately predict weather patterns has never been more crucial. Historically, meteorologists have relied on a combination of observational data and model-based forecasts to predict weather changes. However, with the advent of artificial intelligence (AI) and machine learning, we stand on the brink of a transformative era in meteorological science.
This article delves into the innovative realm where AI intersects with historical weather data, offering a glimpse into how machine learning is not just augmenting but revolutionizing the field of weather prediction. From dissecting complex data patterns to predicting future climatic events with unprecedented accuracy, AI's role in meteorology marks a paradigm shift in how we understand and anticipate the whims of nature. Join us as we explore this exciting frontier, where bytes of data hold the key to unlocking the mysteries of the skies.
The Evolution of Weather Prediction
The journey of weather forecasting is a tale of constant evolution and innovation. In ancient times, predictions hinged on simple observational techniques. People relied on patterns in nature, such as the behavior of animals or the appearance of the sky, to anticipate weather changes. These methods, while rudimentary, laid the foundation for systematic meteorological studies.
With the advent of the telegraph in the 19th century, a significant transformation occurred. This technology enabled faster data exchange and more coordinated meteorological efforts, marking the first steps toward modern forecasting methods. Data could now travel faster than the weather itself, allowing for more proactive planning and response.
The 20th century brought about the era of computer-based models. These models, driven by physical principles, allowed meteorologists to simulate and predict weather patterns with greater accuracy. The use of computers in meteorology represented a paradigm shift, enabling forecasts that were not just reactive but also predictive.
Today, we stand at the threshold of a new revolution, led by Artificial Intelligence (AI) and Machine Learning (ML). These technologies have the potential to analyze vast and complex datasets, something that traditional methods could not handle. This advancement is not just about enhancing short-term weather forecasts; it's about unlocking the potential to understand and predict long-term climatic trends using historic weather data, marking a new era in meteorology.
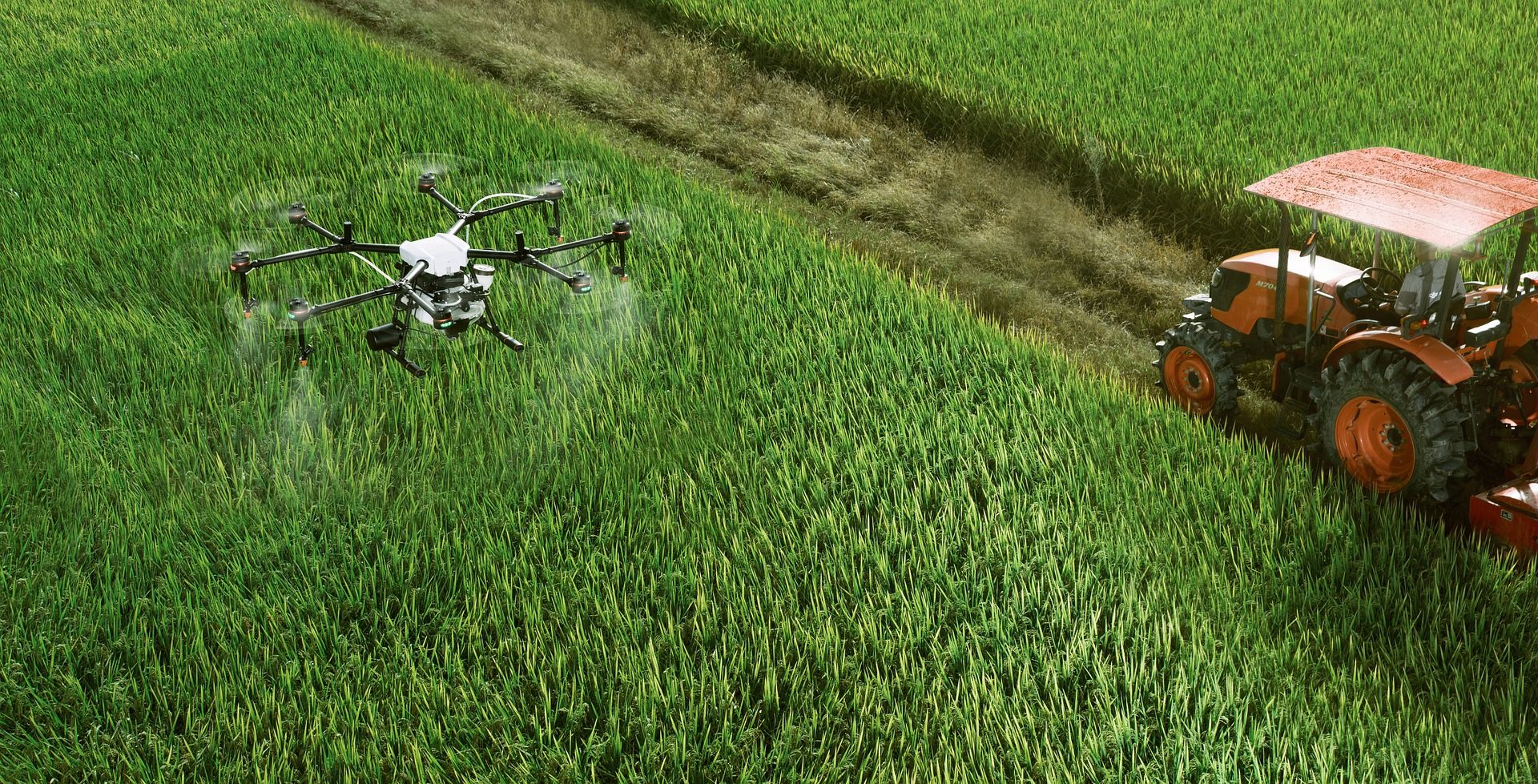
Basics of Machine Learning in Meteorology
Machine Learning (ML) in meteorology is a game-changer, offering tools to decipher intricate weather patterns from historical data. At its core, ML involves algorithms that learn from data, identify patterns, and make decisions with minimal human intervention. In meteorology, this translates to algorithms trained on vast datasets comprising decades of weather information, satellite imagery, and sensor readings.
One key aspect of ML in this field is the diversity of data types. Historical weather data includes temperature, humidity, wind speed, and atmospheric pressure readings. When coupled with modern-day satellite imagery and radar data, these historical datasets provide a rich tapestry for analysis. ML algorithms can discern patterns and correlations in this data that would be imperceptible to human analysts, such as subtle changes in climate patterns over decades.
The commonly used ML models in weather prediction vary in complexity. Linear regression models, though basic, are instrumental in understanding relationships between different weather variables. More advanced models like neural networks and deep learning algorithms offer the ability to process and interpret more complex data structures, essential for capturing the nonlinear and dynamic nature of weather systems.
In essence, the integration of ML in meteorology is about transforming raw, historical weather data into meaningful insights. These insights not only enhance forecast accuracy but also deepen our understanding of weather systems. By leveraging the power of ML, meteorologists are now equipped to build models that can predict not just the likelihood of rain tomorrow but also anticipate long-term climatic shifts, a task that was once considered nearly impossible.
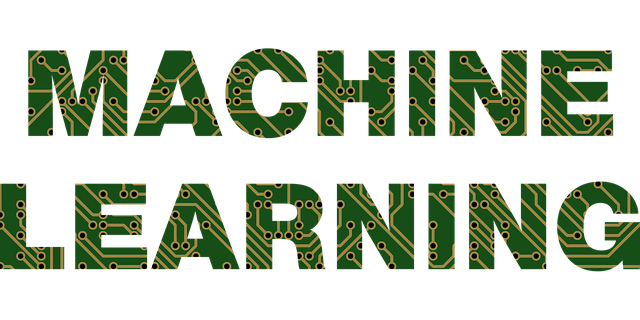
Case Studies and Applications
The practical application of machine learning in meteorology has led to remarkable case studies, each underscoring the potential of AI in revolutionizing weather prediction.
Hurricane Path Prediction
One notable example is the use of ML in predicting hurricane paths. Traditional models often struggled with the accuracy of long-term hurricane trajectories. However, with ML algorithms trained on historical hurricane data, meteorologists have been able to significantly improve the precision of these predictions. These algorithms analyze patterns from past hurricanes, incorporating variables like sea surface temperature and atmospheric conditions, to predict future paths more accurately.
Drought Forecasting
Another area where ML has made a substantial impact is in drought forecasting. By analyzing historical climate data and current soil moisture levels, ML models can predict the likelihood and severity of upcoming droughts. This is crucial for agriculture and water resource management, as accurate drought forecasts can lead to better preparedness and mitigation strategies.
Urban Heat Island Effect Analysis
In urban areas, the heat island effect can significantly impact local climates. Researchers have used ML to analyze historical temperature data, urban infrastructure, and green space distribution to understand and predict this phenomenon. These predictions are vital for city planning and public health, especially in the context of rising global temperatures.
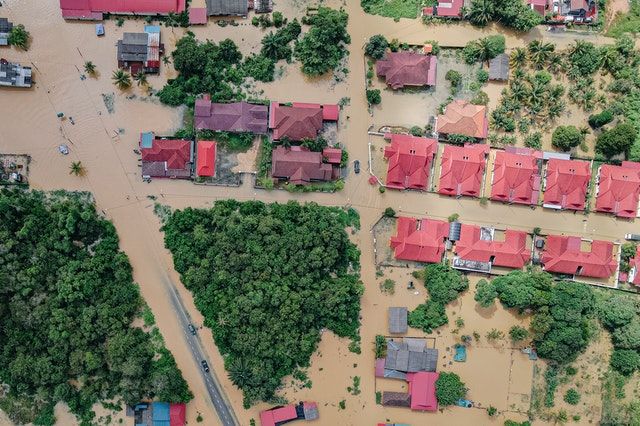
Air Quality Index Prediction
ML has also been employed in predicting air quality indexes. By analyzing historical air quality data alongside weather patterns and pollutant sources, ML models can forecast air quality levels, aiding in public health and environmental policy decisions.
Renewable Energy Optimization
Finally, in the renewable energy sector, ML models use historical weather data to predict solar and wind power generation. These predictions enable more efficient and reliable integration of renewable energy sources into the power grid.
Each of these case studies demonstrates not just the versatility of ML in different aspects of weather prediction but also its profound impact on various sectors dependent on accurate weather forecasts. The integration of AI into meteorology is opening new avenues for research and application, fundamentally altering how we understand and respond to the atmosphere's dynamics.
Challenges and Limitations
Despite the significant advancements brought by machine learning in meteorology, this field is not without its challenges and limitations.
Data Quality and Availability
A major challenge is the quality and availability of historical weather data. ML models are only as good as the data they are trained on. Inaccuracies, gaps, or biases in historical data can lead to flawed predictions. Additionally, in some regions, especially those less developed, the availability of comprehensive and long-term weather data is limited, which can hinder the development and accuracy of ML models.
Computational Complexity
The computational requirements for running sophisticated ML models are another challenge. Processing and analyzing vast datasets, especially when dealing with complex models like deep neural networks, require significant computational power and resources. This can be a limiting factor, particularly for meteorological agencies with limited budgets.
Model Interpretability and Overfitting
Another issue is the interpretability of ML models. Some complex models, like deep learning, operate as "black boxes," making it difficult to understand how they arrive at certain predictions. This lack of transparency can be a problem in scientific contexts where understanding the reasoning behind a prediction is as important as the prediction itself. Additionally, there's the risk of overfitting, where models perform well on training data but fail to generalize to new, unseen data.
Long-Term Predictions and Climate Change
While ML models are excellent for short-term weather predictions and identifying patterns in historical data, their effectiveness in long-term climate predictions is still a subject of ongoing research. Climate change introduces new variables and unprecedented changes that these models have not encountered in historical data, potentially affecting their accuracy.
Ethical and Environmental Considerations
Finally, there are ethical and environmental considerations in deploying ML models. The large energy consumption required for training and running complex models has a carbon footprint that must be considered, especially in a field focused on studying the environment.
Despite these challenges, the potential of machine learning in transforming meteorology is undeniable. Addressing these issues requires continuous research, innovation, and collaboration across various disciplines, ensuring that ML models not only become more accurate but also more efficient and accessible.
The Future of Weather Prediction with AI
The future of weather prediction, augmented by AI and machine learning, holds immense promise. As computational capabilities continue to advance and more sophisticated algorithms are developed, we can expect even more precise and insightful weather predictions. The integration of AI in meteorology is likely to evolve in several key areas:
- Improved Long-term Forecasting: Enhanced ML models, coupled with a better understanding of climate change impacts, are expected to improve long-term weather and climate forecasts, aiding in environmental planning and policy making.
- Real-time Data Processing: The advent of IoT and smarter sensor networks will enable real-time data collection and processing, allowing ML models to provide more timely and accurate weather updates.
- Cross-disciplinary Applications: There will be increased cross-disciplinary applications of ML in weather prediction, combining data from various fields like oceanography, environmental science, and even social sciences to create more comprehensive models.
- Personalization and Accessibility: We may see more personalized weather prediction services, tailored to individual needs and locations, made possible through AI. Additionally, efforts will likely be made to make these technologies more accessible to under-resourced regions of the world.
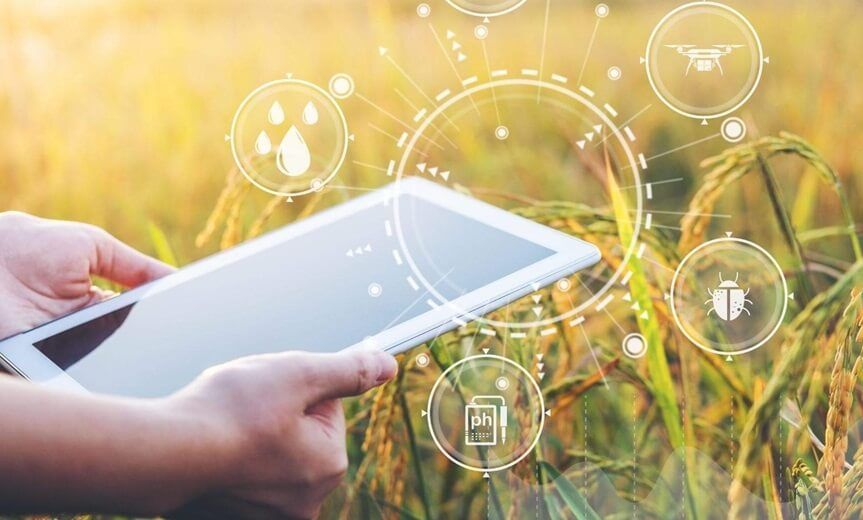
Conclusion
Machine learning's integration into meteorology represents a significant leap forward in our ability to interpret and predict weather patterns. By analyzing and learning from historical weather data, AI has not only enhanced the accuracy of short-term forecasts but also opened new avenues for understanding long-term climatic trends. The case studies in this field exemplify the diverse applications and profound impact of AI in weather prediction, affecting sectors ranging from agriculture to urban planning.
However, as we embrace these advancements, we must also navigate the challenges and limitations inherent in the application of machine learning, such as data quality concerns, computational demands, and ethical considerations. The future of weather prediction with AI is not just about technological advancement; it's about the responsible and sustainable integration of these technologies into our understanding of the Earth's atmosphere.
As we continue to innovate and refine AI applications in meteorology, we stand at the threshold of a new era where the mysteries of the weather are not just observed, but deeply understood, empowering us to make more informed decisions for our planet's future.