Use of AI and Deep Learning in Agriculture
AI and deep learning stand at the forefront of transforming agriculture, offering solutions to enhance sustainability and efficiency amid growing populations and environmental challenges.
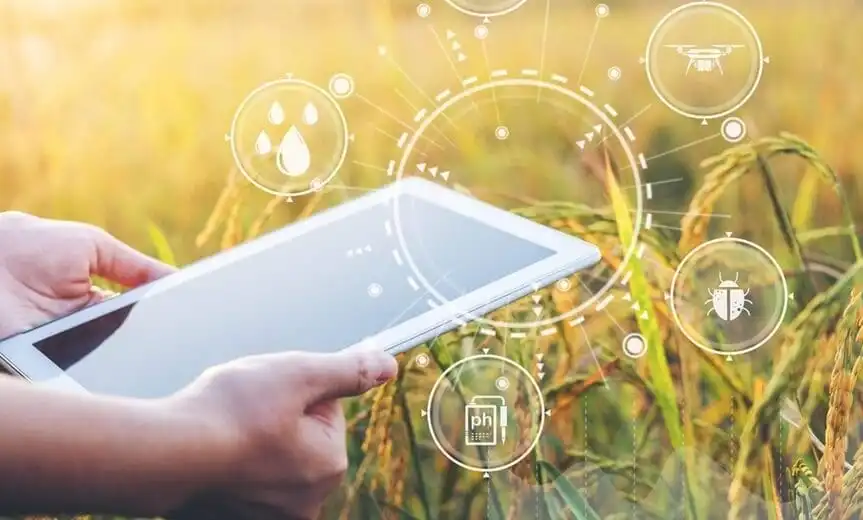
In recent years, the world has witnessed a rapid technological revolution that has permeated every aspect of our lives. Among the countless domains benefiting from this transformative power is agriculture, an industry that forms the backbone of global food production. As the global population continues to grow, and with the increasing challenges posed by climate change and resource constraints, the need for sustainable and efficient agricultural practices has become more pressing than ever. In this context, artificial intelligence (AI) and deep learning have emerged as revolutionary tools, offering immense potential to revolutionize and optimize agricultural systems.
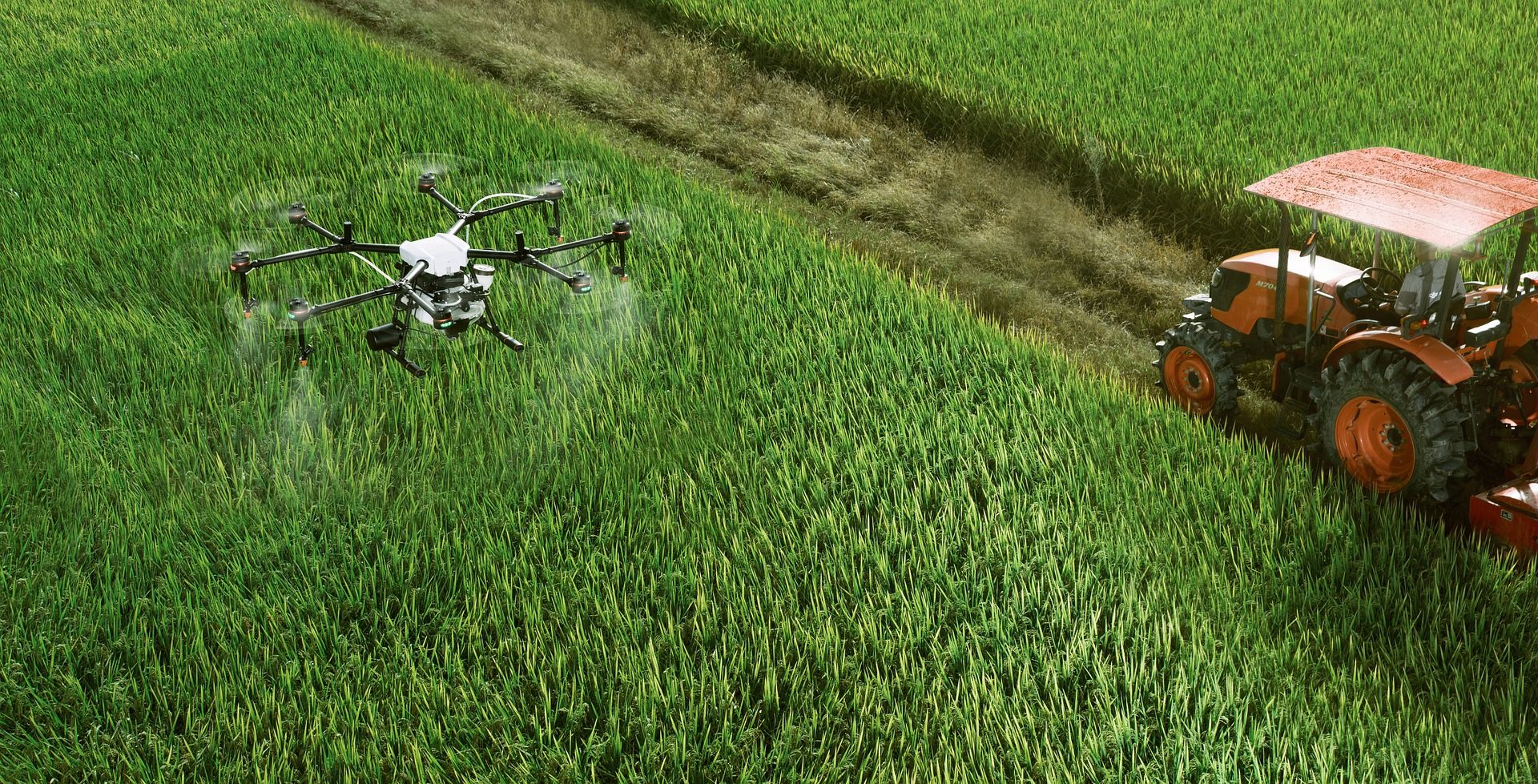
As we embark on a new era of smart and data-driven agriculture, it becomes essential to explore and understand the potential of AI and deep learning to drive innovation, increase productivity, and address the pressing challenges facing our global food systems. By harnessing the power of these cutting-edge technologies, including software for monitoring agricultural fields, we can shape a more sustainable, resilient, and efficient future for agriculture, ultimately ensuring food security for a growing world population. Farm lands monitoring is a cornerstone of modern agricultural practices, made significantly more effective through AI and deep learning integration. These technologies allow farmers to gain real-time insights into the conditions of their fields and crops. Using tools such as sensors, drones, and satellite imagery, farm lands can be continuously monitored for crucial factors like soil health, moisture levels, crop growth, and the presence of pests or diseases.
AI and Deep Learning
Deep learning and machine learning are closely connected fields within the broader domain of artificial intelligence. Machine learning is a branch of AI that focuses on the development of algorithms and models that enable computers to learn from and make predictions or decisions based on data. Deep learning, on the other hand, specifically deals with artificial neural networks, inspired by the structure and function of the human brain. Deep learning algorithms, known as deep neural networks, are capable of automatically learning hierarchical representations of data by progressively extracting higher-level features from raw input. Deep learning has achieved remarkable success in various domains, such as computer vision, natural language processing, and speech recognition.
Therefore, while machine learning provides a broader framework for learning from data, deep learning offers a specialized approach using neural networks with multiple hidden layers to achieve more complex and sophisticated learning tasks. While both deep learning and machine learning are part of AI, deep learning's ability to handle vast amounts of data and extract valuable insights has made it especially useful in agriculture.
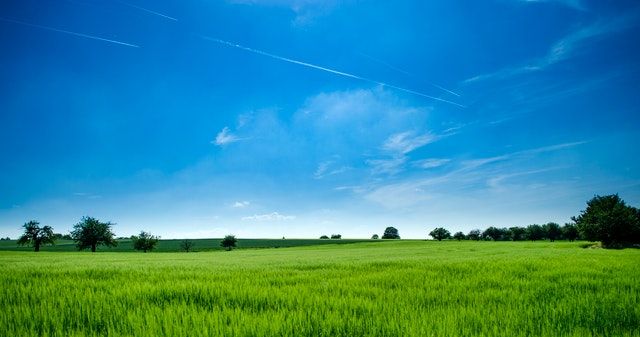
Neural networks consist of interconnected layers of artificial neurons. The input layer receives data, which is then passed through multiple hidden layers, and finally, the output layer provides the desired results. Each neuron in a neural network applies a mathematical function to the weighted sum of inputs it receives. These weights are adjusted during a process called training, where the network learns by iteratively comparing its predictions to the actual outcomes and updating the weights accordingly. This process, known as backpropagation, allows neural networks to improve their accuracy over time.
For instance, EOS Data Analytics is a company that leverages neural networks to provide advanced geospatial analytics and satellite imagery processing. EOS Data Analytics utilizes neural networks for image classification tasks. Data scientists train neural networks on large datasets of satellite imagery to classify different land cover types, such as forests, urban areas, water bodies, and agricultural fields. These networks learn to recognize specific patterns and features in the images, allowing for automated and accurate classification of vast geographic areas.
By training the networks on annotated datasets, EOS Data Analytics can accurately segment different land cover types, vegetation types, or land use categories within a given image. This segmentation data is valuable for tasks like precision agriculture, environmental monitoring, and urban planning.
Deep Learning in Agriculture
Deep learning has found numerous applications in agriculture, enhancing various aspects of farming operations. One notable application is crop yield prediction, where deep learning models analyze historical weather data, soil conditions, and other relevant factors to forecast crop productivity. This helps farmers make informed decisions regarding planting schedules, resource allocation, and market planning.
Another significant application is plant disease and pest identification. By analyzing vast databases of images, deep learning algorithms can accurately detect and classify plant diseases, enabling early intervention and targeted treatment. This reduces crop losses and minimizes the use of pesticides, resulting in more sustainable farming practices.
Furthermore, deep learning models can optimize irrigation systems by analyzing sensor data and soil moisture levels, leading to precise water management and conserving resources. Livestock management also benefits from deep learning, as AI-powered systems can monitor animal behavior, detect anomalies, and provide early alerts for health issues.
AI Market Trends in Agriculture
The integration of AI and deep learning in agriculture has gained substantial momentum in recent years. The market for AI in agriculture is projected to witness significant growth, driven by the increasing need for efficient resource management, yield optimization, and sustainable farming practices. Companies are developing innovative solutions, including autonomous farm machinery, crop monitoring systems, and precision agriculture tools, to cater to the growing demand for AI-powered farming technologies.
Moreover, collaborations between technology companies and agricultural organizations are fostering research and development in the field of AI and deep learning in agriculture. Government initiatives and funding support are further fueling the adoption of these technologies, aiming to achieve food security, reduce environmental impact, and promote sustainable farming practices.
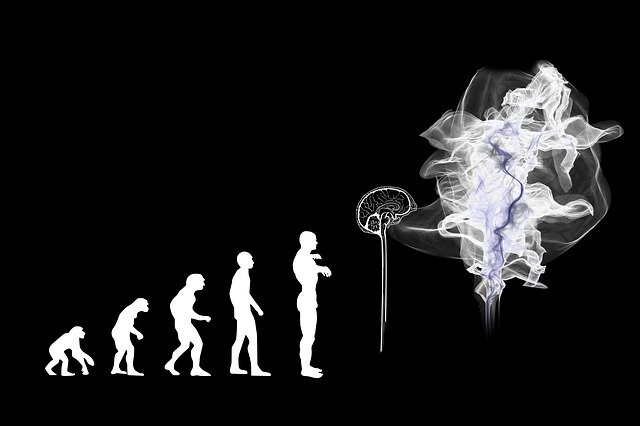
AI and deep learning technologies are transforming agriculture by providing farmers with powerful tools to enhance productivity, optimize resource management, and improve decision-making processes. Artificial neural networks, along with deep learning algorithms, enable the extraction of valuable insights from vast amounts of agricultural data, leading to more sustainable and efficient farming practices. As the agricultural industry continues to embrace AI, the future holds great potential for advanced applications that will further revolutionize the sector, ensuring a secure and sustainable food supply for the growing global population.