How Artificial Intelligence Is Changing Biomedical Research
Biomedical research has been a slow and hectic process involving tireless testing, data analysis, and pattern recognition. AI is making the entire process more efficient and faster, enhancing the potential of groundbreaking discoveries. Explore how AI is shaping the future of healthcare.
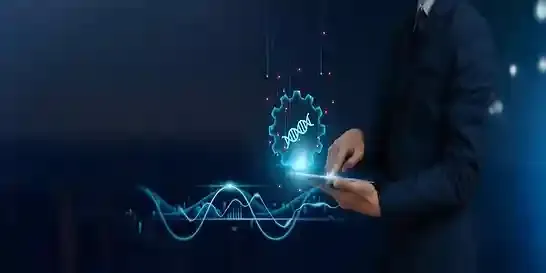
AI is transforming nearly every sector, and biomedical research is no exception. Significant advancements in this field result in massive data collection. Analyzing these complex datasets, such as proteomic or genomics data, is a nightmare for researchers. This time-consuming analysis slows down the progress of a research project. On average, a scientific discovery takes seventeen years to reach the clinic. Here comes AI with its immense potential. It identifies unique trends and integrates data from various sources. Then, it presents a detailed insight that humans can't. AI's ability to process extensive datasets, recognize patterns, and make predictions helps it transform the field of biomedical research. Let's explore the diverse applications of AI in biomedical research, as well as its challenges and prospects.
Role of AI in Biomedical Research
AI in Medical Imaging
Medical imaging and diagnostics are some of the most critical applications of AI in healthcare. Traditionally, a radiologist had to manually examine the X-rays, MRIs, and CT Scans to identify the potential issue. This time-consuming process was susceptible to human error.
Let's have a detailed look at how AI is making an impact in medical imaging:
1. Enhanced Diagnostic Accuracy, Efficiency, & Speed
AI has made disease diagnosis more efficient, quicker, and accurate. ML algorithms analyze medical images to detect abnormalities like tumors, lesions, fractures, etc. It can recognize the patterns that a human eye can’t, hence automatically segments and labels the organs and abnormalities in medical images. It reduces the time and effort required for manual analysis. AI models have shown great promise in identifying the early symptoms of diseases like cancer, and cardiovascular and neurological disorders even before the symptoms appear. AI tools like Freenome, Visual Dx, ENDEX, and IBM Watson highlight the areas where doctors need to focus more. Leveraging advanced deep learning algorithms, these AI tools assist healthcare professionals in the initial screening process.
2. Automation & Cost Efficiency
AI automation has reduced the burden on radiologists and other healthcare professionals. They can now concentrate on more complex tasks than image processing, quality control, and data management. Task automation tools like Rad AI Impressions and AI chatbots have also reduced the need for many specialists, making AI more cost-efficient, especially in developing areas.
3. 3D Imaging and Reconstructions
AI helps create 3D reconstructions of medical images. These 3D HD images of anatomical structures are crucial for surgeries and understanding complex conditions.
AI in the Discovery & Development of Drugs
The job of a biomedical researcher is tiresome. They work hard to uncover the disease mysteries and develop life-saving drugs. AI offers innovative solutions to the challenges that typically slow down drug discovery and development. It contributes significantly to discovering new drugs, particularly in the context of Antimicrobial Resistance (AMR), which has posed a global threat. Here are some key ways AI is backing the field.
1. Target Identification
AI analyzes large biological datasets and identifies the targets. The targets are the biological molecules, such as proteins or genes, mainly responsible for disease occurrence. The techniques, such as genomic approaches, proteomics, bioinformatics, or screening and imaging, help identify targets.
2. Target Validation
Once the potential target is found, it is passed through various experiments for validation. The experiments, including gene knockouts, biological assays, or overexpression studies, confirm if the target is involved in disease occurrence and its modulation will have a therapeutic effect. Hence, the potential drugs focusing on a validated biological molecule are discovered.
3. Virtual Screening & Compound Optimization
AI models filter the vast library of chemical compounds and predict the compounds interacting more effectively with the biological target. The most promising compounds then undergo the optimization process. AI models like GANs and VAEs are used to generate novel materials with desired properties. Compound optimization enhances their properties, including binding affinity, stability, and safety. Together, these techniques help find effective therapies to speed up drug discovery.
4. Drug Toxicity Prediction
Prediction of a new drug's toxicity and side effects is crucial before testing it for clinical trials. Here again, AI helps. The most used AI models for drug toxicity prediction are Tox21, ChEMBL, ICE, and hERGCentral. The researchers use AI and computational models to predict if the new compounds may negatively impact the body. Predicting adverse reactions early in the development process reduces the risk of late-stage failures and ensures safe drugs for the patients.
5. Accelerating Clinical Trials & the Availability of Drugs To the Clinic
Before starting the drug discovery process, the researchers must test more than 100,000 potential candidates. AI helps narrow the candidate pool and accelerate this process by identifying suitable patients for clinical trials. Biomedical researchers can now optimize trial designs and analyze data more efficiently.
AI in Understanding Biological Complexity
The human body has 37 trillion cells. Each cell contains around 20,000-25,000 genes and up to 10,000 proteins, which interact millions of times per second to help the cell perform a distinct genetic activity. These interactions, which occur in a dynamic, interconnected network, are very complex. Understanding this biological complexity demands the collection of vast data and the identification of its patterns. Here comes AI to facilitate the process.
Let's explore how AI is stepping in here as a crucial tool.
1. AI ensures Data-Driven Insights
With AI, researchers can interpret massive datasets collected over decades. This data-driven approach enables scientists to gain deeper insight into the human body’s functions by identifying new patterns and the relationships within cellular systems.
2. AI Improves Hypothesis Testing Efficiency
Hypothesis testing is a crucial step in the scientific method for biomedical research. It allows the researchers to validate or reject a proposed theory. For hypothesis testing, the researchers at Columbia University have developed an open-source AI model called GET (General Expression Informer). Using this AI tool, the researchers can stimulate molecular interactions using the "in silico” (a computer model) drug design method and test a hypothesis. It saves time and money on laboratory experiments. Hence, scientists can test several hypotheses in the minimum time possible and identify the most promising areas for further study.
3. AI is Uncovering Universal Biological Principles
All human cells share a specific pattern in their function, which is usually hard to recognize. Here again, AI helps scientists understand each cell's behavior by identifying the patterns of molecular activity in different cells. Hence, scientists can find common principles of cell behavior. Just like Newton's law of Physics gives us some basic rules to understand the movement of objects, AI models uncover the basic rules of cell behavior. Hence, the scientists can better understand the body functions and disease occurrence.
4. AI Helps In Exploring Epigenetics & Cellular Behavior
Epigenetics studies how genes turn on and off to influence cellular function. AI is revolutionizing the study of epigenetics by analyzing massive biological datasets and simulating molecular networks. AI models like GET predict how proteins and other molecules interact and influence each other in a cell. Hence, AI helps scientists in the following ways.
- Studying cellular processes like Differentiation.
- Identifying key nutrients that regulate gene expression.
- Understanding how cells respond to signals like hormones or toxins.
- Predicting how molecular changes, such as mutations, could disrupt normal cell functions, leading to diseases like cancer.
Enhanced Treatment Planning & Personalized Medicine
AI's ability to do data fusion can help combine different medical images, e.g., MRI and PET scan data, etc. Hence, healthcare professionals can have a comprehensive view of patients' conditions. Moreover, the quantitative evaluation of the radiographic traits further helps assess disease progression and treatment response. AI image analysis also helps doctors plan personalized treatments, get real-time feedback, and monitor an abnormality's exact size and position.
AI integrates pharmacogenomics data to analyze how genes affect a person's drug response. This integration helps researchers predict a patient's response to a particular medication and recommend personalized drug regimens. It improves the effectiveness of the treatments and reduces the risks. AI in healthcare also helps create customized treatment plans based on medical history, lifestyle factors, and real-time health data.
Discovering New Insights Into Diseases
Unlike traditional methods, which are inefficient in handling complex datasets, AI can analyze large-scale genetic, proteomic, and molecular interaction data. Its ability to identify biomarkers, i.e., the biological indicators of the presence and progression of the disease and its response to treatment, promotes deeper insights into disease mechanisms. AI algorithms recognize patterns and relationships in the data to reveal hidden insights.
Let's look at some diseases AI has contributed to understanding and treating.
Alzheimer’s Disease
AI tools analyze vast data to uncover genetic mutations and molecular mechanisms contributing to Alzheimer’s disease. By processing the data obtained from genetic sequencing, protein interactions, and brain imaging, AI highlights the most contributing factors for disease occurrence and progression.
Pediatric Leukemia
This is a type of cancer in children where AI has made valuable insights. By leveraging AI models, the researchers have analyzed how genetic mutations in the DNA affect the interactions of proteins and other molecules, disrupting normal cell functioning and contributing to the development of leukemia. It has helped biomedical researchers formulate new strategies for targeting those identified molecular changes, leading to refined treatment options and patient outcomes.
Infectious Diseases
AI is helping to accelerate the process of genomic surveillance, which is crucial for understanding the evolution and transmission of infectious pathogens. From detecting outbreaks to accelerating vaccine development, AI plays a pivotal role in contagious disease biomedical research. Its contribution to tracking the evolution of viruses, such as SARS-CoV-2, by analyzing the genomic sequence of different variants is impressive. During the COVID-19 pandemic, AI applications did wonders in predicting its spread, combating it, and vaccine development. AI models can easily predict the parts of a pathogen's genome that are more likely to be effective vaccine targets. By simulating immune responses, AI helps to optimize vaccine formulations, eliminating the time-consuming trial-and-error testing.
Challenges of AI in Biomedical Research
Although the benefits of leveraging AI in biomedical research rapidly transform the biomedical landscape, several challenges must be addressed.
1. Lack of Transparency
Though AI accelerates the entire biomedical research project's conclusion, researchers find it hard to understand those conclusions. If a doctor does not clearly understand how a prediction was made and how a diagnosis was reached, he would not be able to make an informed decision. Moreover, how could he gain a patient's trust without explaining the background? This limitation, often called the "Black Box" problem, can hinder adoption of AI in some contexts.
Promoting the use of Explainable AI systems helps improve AI's transparency and interpretability, as they focus on devising methods that can be understandable to humans. Using interpretable models like decision trees instead of complex models like neural networks can help cope with the transparency challenge.
2. Biased Data
AI models are often trained on datasets representing a specific group of people (say, specific age, race, or health condition). This model will not work well when applied to a diverse population. So, if an AI model trained on data from young adults is used for older adults, it will cause bias and could give unfair or incorrect results.
AI models must be regularly updated with new and diverse data to stay relevant and accurate across diverse populations. Moreover, implementing fairness and techniques like "adversarial debiasing" or "re-weighting data" can reduce bias during training.
3. Regulatory Compliance/Data Privacy Concerns
Biomedical research often involves sensitive patient data protected by laws like HIPAA in the United States. Since AI requires massive amounts of data to learn and make accurate predictions, it can pose significant risks (like data breaches) to an individual's privacy if not appropriately handled. AI models need protection against cyberattacks or accidental leaks of sensitive health data, as data breaches could lead to significant harm and loss of trust.
AI tools, especially those used for diagnosing diseases or recommending treatments, must be FDA-approved or comply with similar regulatory bodies. AI-driven treatments must undergo rigorous clinical trials and follow the regulatory approval process.
Future Prospects
AI has emerged as an incredible partner in biomedical research, revolutionizing how scientists tackle complex medical problems. The future of AI in biomedical research is bright with the continuous advancements in AI algorithms, data integration, computational power, and interdisciplinary collaboration. It opens new avenues for discovery, diagnosis, and treatment that were unimaginable earlier. These breakthroughs are only the tip of the iceberg. By overcoming challenges, Artificial Intelligence will drive significant advancements in the biomedical research segment. With continuous advancements, the next milestone will be predicting cellular changes before they happen– the development that would push personalized medicine into prevention.
As Raul Rabadan, a system biology professor at Columbia who is a prominent figure in the field of biomedical research and famous for his work at the intersection of AI in biology, says:
“We are entering in an exciting era. Biology is being transformed into a predictive science.”