Security and Data Annotation: Ensuring Your Data is Safe with Your Annotation Provider
In our latest blog, we delve into the critical intersection of security and data annotation, highlighting the paramount importance of safeguarding your data when partnering with an annotation provider. Learn how to ensure your valuable information remains secure throughout the annotation process.
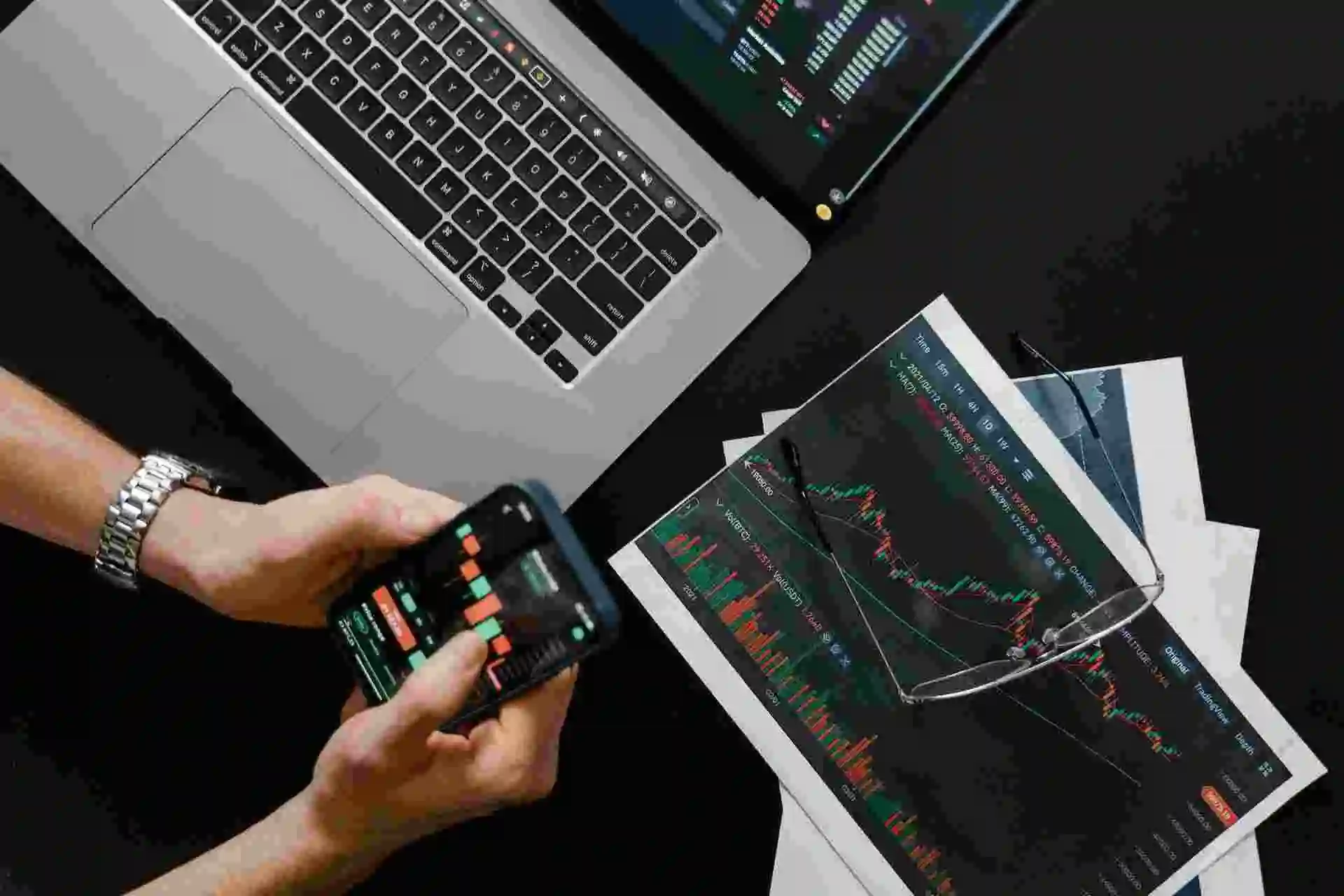
When your data is no longer in your control, what happens to it? There is always a possibility of data mismanagement and unwanted access due to this reliance on external annotation suppliers. Having your annotation service provider guarantees the safety of your data. This article delves into the important topic of data security in the context of data annotation and discusses the steps you can take to keep your data secure when you annotate it.
Annotating Data: What Is It?
Annotating data may sound like an impossible task, but it's really just a way to give computers the same ability to understand and interpret the world as humans do. AI data annotation adds value and insight to raw data by meticulously labeling, tagging, or marking data elements. This process is vital for any AI developer looking to train accurate and effective models. To make sure we're all on the same page, let’s review the fundamentals of data annotation.
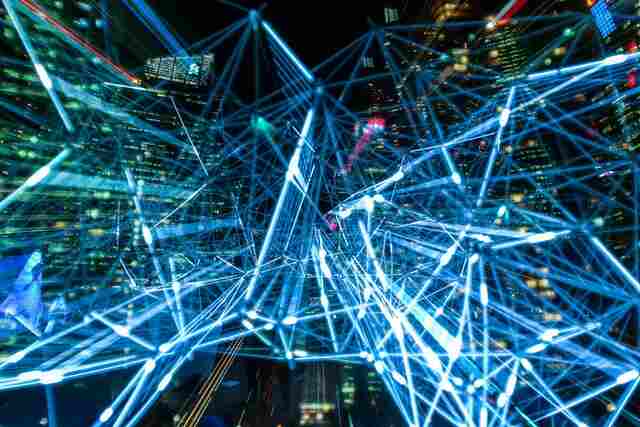
Types of Data Annotations
- Image Annotation: When it comes to computer vision, picture annotation is king. It's a visual feast for AI, with bounding boxes and polygon segmentation helping machines recognize objects and their properties within photographs.
- Text Annotation: Annotating text is essential in the field of natural language processing. The foundations of textual data analysis are found in NLP techniques such as named entity recognition, sentiment analysis, and part-of-speech tagging.
- Audio Annotation: Audio annotating is essential for people who want to use machine learning to train speech recognition or sound classification models. The ability to understand auditory data is facilitated by methods such as phoneme alignment, transcription, and labeling of audio events.
- Video Annotation: Object tracking, action recognition, and the annotation of time-based events are all part of the ever-evolving field of video annotation. It's a must-have for any video analysis system powered by AI.
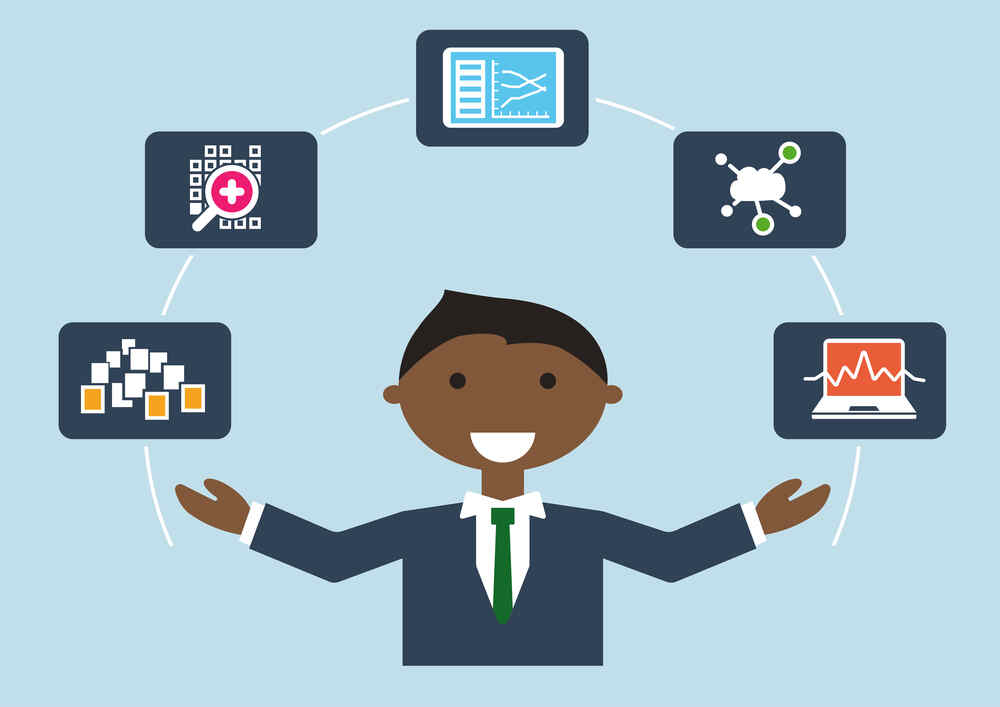
Importance of Data Security
When evaluating potential outsourcing partners for remote data annotation projects, make sure to take data security and privacy into account. Over 90% of CEOs and CTOs have already begun to invest in AI and machine learning. Unfortunately, the cost of technical progress is that more than 62% of businesses have difficulty meeting the requirements of data legislation like GDPR and CCPA.
Data privacy and security are becoming increasingly important as our reliance on technology grows. This is understandable in light of the numerous high-profile data breaches that have occurred over the past several years.
Common Security Threats in Data Annotation
Data annotation services pose a number of risks to data privacy and integrity.
- When employees use a public Wi-Fi connection or an unprotected computer, your data is at risk.
- Certain pieces of your data may be downloaded and saved by employees.
- Screenshots can be taken and sent out through several channels.
- If a data annotation specialistsits in a public area and labels your data.
- A lack of awareness, understanding, and accountability among workers has compromised security.
- Low-quality data produced by human mistake during the annotation process can negatively impact the effectiveness and reliability of AI and ML models. According to research conducted by Gartner, businesses might lose up to 15% of their income due to poor data quality.
A data security certification like HIPAA or SOC 2 is lacking from your labeling service.
Best Practices for Data Security in Annotation
Let's look into the most effective methods for protecting annotated data, including encryption, frequent security audits, personnel training, tool selection, and access restriction.
- Encryption: The first step in data security is to safeguard data when it is not being directly processed. To prevent unauthorized access, secure annotation platforms use strong encryption methods like AES-256. Database information is protected against theft even if the server itself is taken because of this encryption.
- Regular Security Audits: Weaknesses, gaps, and improvement potential are all exposed by these audits. After that, recommendations for improved data security might be made by security professionals.
- Employee Training and Awareness Programs: Personnel should have a thorough understanding of data security protocols. Consistent training sessions inform them of the most recent dangers, how to spot phishing attempts, and the value of secure password management. When a culture of security is fostered, workers take the initiative to safeguard private information.
- Using Secure Tools: Safe annotating software has safeguards by design. They are up to date with the latest security patches and follow established norms for protecting sensitive information. Always opt for well-respected tools with a solid track record of safety.
- Multi-Factor Authentication: The use of MFA is like adding armor to a door. It necessitates the presentation of multiple forms of user authentication, such as a password and a physical possession (such as a cell phone). Even if a password is compromised, MFA provides a strong barrier to entry.
- Strict Access Controls: Access to sensitive information is restricted to just a select few. The goal of role-based access control (RBAC) is to limit users' access to data that is directly related to their assigned roles. Organizations can reduce the possibility of data leaks, both inadvertent and malicious, by limiting who has access to sensitive information.
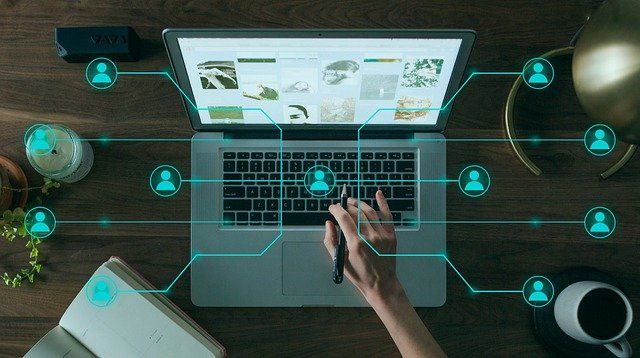
How to Find a Data Annotation Service Provider?
Outsourcing data annotation and dataset labeling requires the same diligence as the outsourcing of any other mission-critical service. It's important to weigh the pros and cons of various data annotation companies by learning more about their backgrounds and the results they've achieved for previous clients. While cost should always be considered, going with the absolute lowest option could lead to frustration and wasted time if the service doesn't live up to expectations.
A proof-of-concept (POC) dataset can be used to conduct parallel tests on multiple service providers to determine which one performs the best. Using this strategy, you may compare and contrast the annotations from various service providers in terms of quality and precision.
In conclusion
Finding a reliable company to handle your data annotation needs can be challenging. However, if you take into account the aforementioned aspects, you can choose a data annotation service provider that meets your needs in terms of quality annotations, tailored solutions, and outstanding support—all without breaking the bank or your deadline.