Data2vec: Meta Released First Multitask Learning Algorithm
Recent advances in AI are continuously being announced, however each is concerned to a certain domain. Researchers are working to make AI more adaptable than ever before. Data2vec integrates the multitask learning process and performs three tasks at once: recognizes pictures, speech, and text.
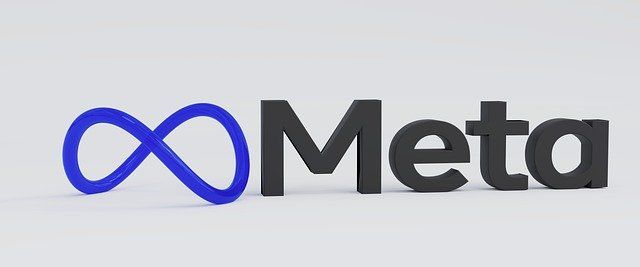
The fast-paced technology creates a multitask learning neural network with the ability to process multiple tasks simultaneously. A more generalized artificial intelligence that works above data discrimination and crunches them all under a basic instructional frame.
The global market size of machine learning was $11.33 billion in 2020. According to Fortune Business Insights, the machine learning market is expected to grow from $15.5 billion in 2021 to $152.24 billion by 2028 with a 38.6% CAGR. The stats show its potential of changing and benefiting the world in the future.
Recent advances in the AI space are coming out constantly, but every advancement is limited to a specific domain. For instance, a method used to produce synthetic speech cannot recognize facial expressions. Meta, formerly Facebook, researchers want to change it. They are struggling to make artificial intelligence more versatile than ever, producing a system that can learn on its own capabilities whether it is about speaking, writing, or viewing something. Data2vec algorithm unifies the multitask learning process and performs three tasks simultaneously; recognize images, speech, and text. A researcher at Meta AI, Michael Auli, said that this algorithm would change the thinking behavior of people about performing multitask learning by machines.
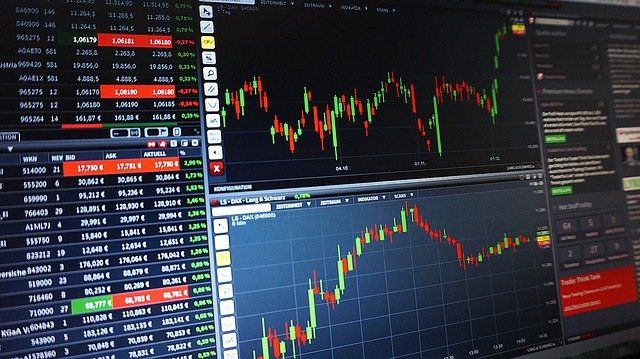
1. WHY DID META DEVELOP THE SSL MODEL?
The traditional way of training the artificial intelligence model was comprised of introducing millions of labeled examples of a specific object or element. For instance, a facial recognition system cannot recognize speech or generate textual content, and a credit card fraud detector cannot detect cancerous patients. Traditional machines are confined to a specific niche; machines' AI prowess was not transferable. But this approach is no more feasible as researchers found it tedious to manually create databases (as large as required to train algorithms of next-gen AIs).
Currently, one of the most promising AI technologies is self-supervised models that work from prominent quantities of unlabeled data, like videos and books presenting people interacting. And consequently, build their understanding regarding the rules of the system. for instance, these models read thousands of books and understand the relative position of words about grammatical structure (objects/articles/commas) without human interference.
This shortfall in the AI industry raised a question in Meta intelligence researchers' minds to develop data2vec with the capacity to outperform current processes of various same-sized models.
If one can identify a dog after seeing it, it must be possible to explain it in words. The given phenomenon is no longer limited to humans now as AI is surprising similarly. Deep neural networks are now efficient at identifying elements in photos and communicating via natural language, but separately. The artificial intelligence models have excellence in one trait but not both.
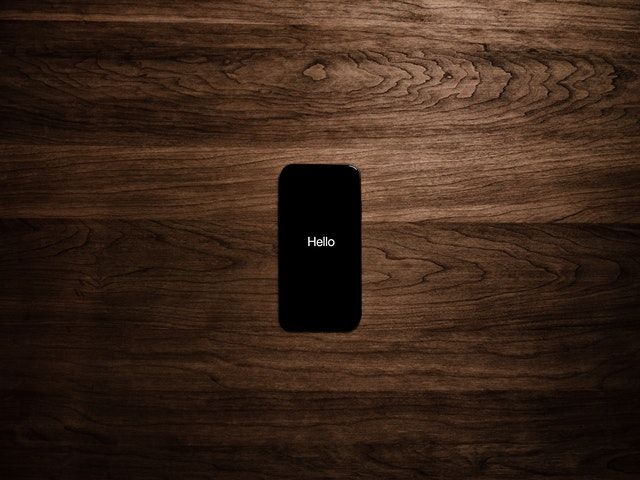
Before knowing what data2vec is and how it is constructed, let's discuss some important parameters to understand a meta concept and its uses better.
2. WHAT IS META?
Meta is defined as a level above. It raises the stage of abstraction one step and shares information about something else. For instance, meta-data stands for data about data, typically explained by metadata. Data is stored in a file, and the example representing metadata is data about that data stored in files like its name, size, type, date created, date modified, and path. Let's discuss the use of the term meta in machine learning, known as meta-learning.
3. WHAT IS META-LEARNING?
Meta-learning is defined as learning about learning. In terms of machine learning, learning refers to learning algorithms as an output of other ML algorithms. Further deeper, ML algorithms learn from historical data. For instance, SL (supervised learning) algorithms map different input patterns to different output patterns for solving classification and regression predictive modeling issues.
Historical data is used to train algorithms to develop a model. That prepared model is then applied to predict the output of a specific situation. Algorithms can be classified as learning algorithms and meta-learning algorithms based on meta-involvement.
Learning Algorithm: Make new predictions based on learning from historical data.
Meta-Learning Algorithm: ML algorithms need the output of other ML algorithms to learn from their data. It means they require other learning algorithms that are already trained. In this way, meta-learning is one step ahead.
4. WHAT IS META-LEARNING USED FOR?
Meta-learning is a potential area of research that learns how to deal with problems. The answer to a meta-model in machine learning is the development of models that can learn skills quickly and modifiable to a new environment with the least training examples. It improves the learning speed and the design of neural architectures and offers novel approaches to be learned simultaneously. Meta-learning is set to train a model on a diverse set of information to solve new problems with a handful of training examples. It focuses on agnostic modeling with multitask learning that is deeply concerned with model architecture. The meta-learning algorithms make intelligent systems with quick multitask learning, adaptable to changes, and generalized to many tasks.
5. DEVELOPMENT OF DATA2VEC, META ALGORITHM
Facebook learning and development researchers used a self-supervised learning strategy where neural networks learned to spot data patterns set by themselves. Here, they do not guide the algorithm through labeled examples. Large language models, including GPT-3, are trained in the same way a wide variety of unlabeled data was scraped from the internet and made deep learning more advance than ever. Auli and his colleague worked on self-supervised teaching AI to train an algorithm for voice recognition at Meta AI. They looked at other co-workers doing a similar process with self-supervised learning to train it for texts and images. All of them were using different techniques to achieve the same goal.
Data2vec is declared the first high-performance self-supervised model accessible by multiple modalities. It is found to be competitive on natural language processing tasks as it does not rely on contrastive learning or does not demand updating input manually. The company also issued its open-source code and pre-trained models.
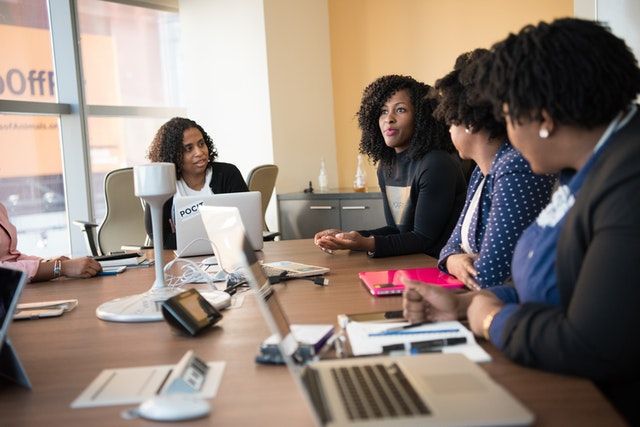
6. HOW DATA2VEC WORK?
Research in SSL (self-supervised learning) is focused on one specific modality in recent works. So, researchers working on one modality use a different approach than those working on another modality. For instance, speech models are trained on an inventory of basic sounds to predict the missing sound rightly. In text algorithm, researchers train models to predict the right word in the blanks of the sentences. Vision algorithm is trained to identify similar images of the same representations in the vision.
Moreover, algorithms predict separate units for different modalities; words for text, pixels, or visuals for images, and learned sound inventories for speech. Pixel collection is quite different from the passage of text and audio waveform. It means that algorithms must function differently in each case.
Meta published a paper, namely, Data2vec: A general framework for self-supervised learning in speech, vision, and language. Data2vec simplifies the issue mentioned above by training models to predict their own input data representation (eliminating modality). A single algorithm can deal with different types of input data. This approach ends the dependence on modality-specific targets in given tasks. However, it is not straightforward to directly predict presentations (neural network layers).
The team said they trained this first algorithm by giving a partial view of the input data. They explained in the paper that they used two neural networks in Data2vec- a student and a teacher. The student mode learns from the teacher mode and updates the model parameters in real-time. First, they trained the teacher network in the usual way on images, speech, and text and allowed it to learn from the internal presentation of data. Then it is asked to predict what it is seeing when tested with new examples. It recognized the dog's photo when tested with the picture of a dog.
The twist was here when they trained student neural networks to predict the internal representation of the teacher model. In simpler words, it is not trained on the image of a dog but to guess what the teacher network sees when shown that (dog) image. Data2vec is a clever idea; the student doesn't guess the actual image or a sentence but the representation of teacher neural network, the algorithm does not to be trained on a specific input-type. Meta AI researchers trained the model on 960 hours of audio, images from ImageNet-1K, and many thousands of books, including Wikipedia pages. It is a promising technique in generalized systems for multitask learning.
Baevski, a Meta AI researcher, dreams of introducing special applications of the metaverse. He said this technology would build AR glasses with an AI assistant. It would help cooking food, notice if any ingredient is missing, and turn down the heat when required with other complex and brilliant tasks. He said:
Imagine having a model that has been trained on recordings of thousands of hours of cooing activity from various restaurants and chefs. Then, when you are cooking in a kitchen wearing your AR glasses that have access to this model, it can overlay visual cues for what you need to do next, point out potential mistakes, or explain how adding a particular ingredient will affect the taste of your dish.
6.1. Architecture
The team used:
- Standard Transformer architecture with input data encoding (modality-specific) taken from previous work. Unlike other transformer-based models like OpenAI’s GPT-S and Google's BERT, data2vec focuses on inner neural network layers representing data before producing the final output. It doesn't create various output data types before generating the final output. It happens due to the self-attention mechanism of a self-supervised system that allows input to interact.
- ViT image encoding strategy as a sequence of patches spanning 16û16 pixels, input for a linear transformation. Alexey Dosovitskiy develops a vision transformer neural network at Google for visual applications.
- Then they encoded speech data with a multi-layer 1D convolutional neural network. It maps waveform from 16kHz to 50kHz.
- Sub-word units are obtained via pre-processing the text. Learned embedding vectors were used to embed it in distributional space.
6.2. Masking
The input samples are embedded as a sequence of tokens, and after it, the team masked a portion of these units by replacing them with a learned mask embedded token. Finally, they fed the sequence to the Transformer network.
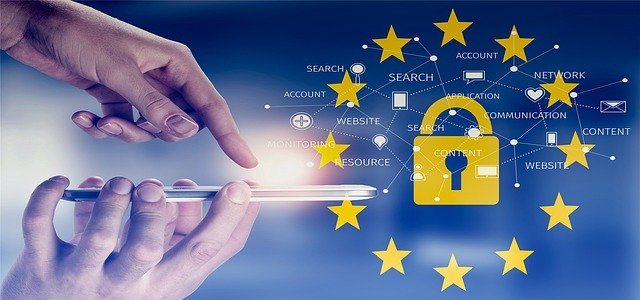
6.3. Computer Vision and Language
Now they used Bao's (2021) strategy for block-wise masking and masked tokens for languages.
They trained the model to predict the model's representations of the original training sample (unmasked) based on the masked sample encoding.
6.4. Results
Testing of data2vec showed that it was competitive with and surpassed other similar-sized exceptional models. For instance, if all models are limited to being 200 megabytes, data2vec showed better output.
- Computer Vision: They tested the method on the ImageNet-1K training set, and they found it resulted in a model fully tuned for image classification by using the labeled data of the same standard. It surpassed similar present strategies for famous model sizes.
- Speech: Their model surpassed HuBERT and wav2vec 2.0; both are Meta’s self-supervised algorithms for speech recognition.
- Text: Data2vec was tested on the GLUE benchmark and gave outperformed results on par with RoBERTa.
7. AFTER ALL IS SAID AND DONE
The data2vc idea was to build an intelligent framework that could learn abstractly. It means it starts from scratch (performance depends on how vast data is given for learning). One can give it books to read, speech to sound, and images to scan, and after a little learning, it is here to perform multiple tasks. It is just like starting with a single seed, and the grown food quality depends on the fertilizers you give to the soil.
Meta's team wrote in a blog that creating data2vecis to learn more generally. Artificial intelligence high-performance work systems should perform multiple tasks, including entirely different ones. Data2vec will bring the coming generations closer to a world where computers are given with least labeled data to outperform tasks. Mark Zuckerberg said in this post that in this way, people understand the world as a combination of vision, speeches, and words, and one day artificial systems will understand the world like humans.
However, this research is an early stage of machine efficiency, so don't expect it too easy to merge general AI suddenly. AI with a generalized learning structure works well with various data types. It offers better and more elegant solutions in one model than the fragmented intelligent models. Multi-model systems need much attention to the data to achieve efficiency; for instance, OpenAI’s CLIP model mistakenly classifies the image of apple as an iPod if the word “iPod” appears in an image. And same uncertainty could be found in data2vec. Baevski said:
We have not specifically analyzed how our models will react to adversarial examples, but since our current models are trained separately for each modality, we believe that existing research on adversarial attack analysis for each modality would apply to our work as well.