AI in Radiology
Deep learning algorithms create neural networks with several hidden layers, allowing them to generate more insights than linear algorithms. These are used to create high-quality medical images. In terms of processing medical data and deriving meaningful insights, AI in radiology is a natural match.
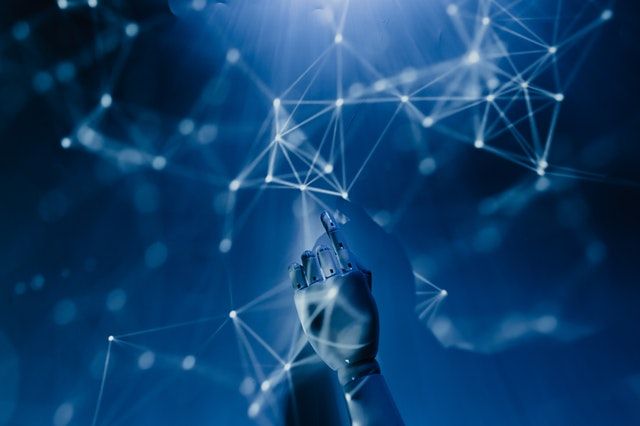
The radiology department in healthcare is likely to search for improvements. 45% of radiologists experienced burnout even before COVID-19 in their careers. The researchers felt exhausted with the administrative burden and manually checked a large number of images. The number of scanning images may reach over a hundred scans a day. Furthermore, this industry lacks non-invasive tissue classification techniques, while invasive procedures are time-consuming, causing stress in patients. In this scenario, AI jumps in, providing the healthcare solutions to rescue. In 2018, the global AI radiology market was expected to grow at $21.5 million and reach $181.1 million by 2025 with a CAGR of 35.9%. According to Absolute Market Insights, the AI in radiology market will reach $3506.55 million by 2027 globally. However, with various advantages of AI in radiology, challenges still influence its widespread.
1. What IS AI, AND HOW IS IT USED IN RADIOLOGY?
AI has the potential to create intelligent applications and machines that mimic the cognitive functioning of humans, like learning and problem-solving capabilities. Researchers apply machine learning algorithms in radiology by training them in lungs scan. Deep learning solutions develop neural networks with multiple hidden layers to drive more insights than linear algorithms. These algorithms are used to generate medical images with enhanced quality. AI in radiology is the perfect match in handling medical information and extracting significant insights.
2. UTILIZATION OF AI IN RADIOLOGY VIA TWO WAYS
- Experienced radiologists supply predefined criteria for properly programming an algorithm. These rules are introduced into the software for performing clinical tasks.
- It allows algorithms to learn from a large volume of data with supervised or unsupervised strategies. The algorithm is trained to extract information via patterns and share deep insights.
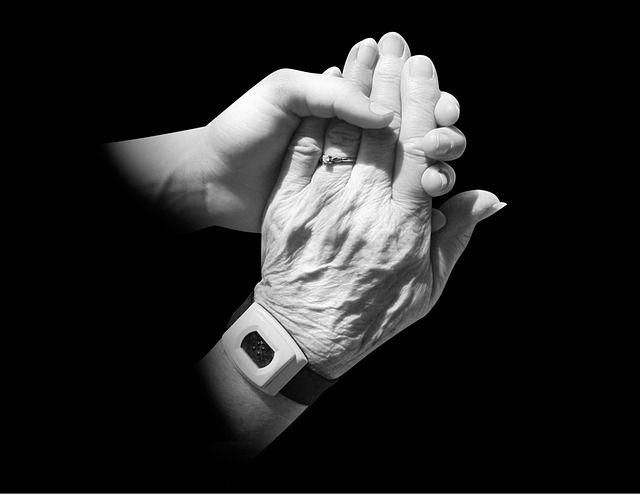
3. ARTIFICIAL INTELLIGENCE RADIOLOGY COMPANIES
Here is the list of companies utilizing the potential of AI in medical imaging to share an incredible experience of healthcare services.
1. IBM Watson (Country: USA)
It is one of the exceptional radiology AI companies serving the healthcare department with artificial intelligence to deliver successful solutions in several ways. The company is committed to offering fast processing medical images and interpreting data files in real-time with information from several databases.
2. Butterfly Network (Country: Mexico)
The company strives to introduce a new technology perspective in medical imaging with hardware and software solutions. Its portable mobile device, Butterfly IQ, uses UOC (ultrasound-on-chip) technology, making it the first to handle the entire body scanning framework. The Chairperson of Butterfly Network, Dr. Jonathan Rothberg, is a recipient from the White House dealing with the National Medal of Technology and Innovation.
3. Arterys (Country: USA)
It developed the first product to visualize and quantify blood flow in the body via MRI (magnetic resonance imaging). Moreover, it received FDA approval for clinical cloud computing service in the healthcare department and was awarded in 2016 as "The Best New Radiology Vendor" and "Best New Software." Fast Company ranked it among the world's 50 most innovative companies in 2019. Plus, its lung-AI platform reduces missed detections up to 70% from 42%.
4. Gauss Surgical Inc. (Country: California)
The company developed the world's first and only mobile app for real-time monitoring surgical blood loss. It also received Conformite Europeenne (CE) Mark for its iPad-specific Triton System.
5. Zebra Medical Vision (Country: Israel)
Israel-based company is considered one of the most innovative companies in 2017 in the machine learning sector. Plus, it received FDA approval in 2019 for producing the world's first chest x-ray AI triage product.
6. Aidoc (Country: Israel)
It is one of the radiology artificial intelligence companies that supports and enhances the radiologist's diagnostic impact power with AI-supported intelligent solutions. The company aids expedite patient treatment and offer improved quality health care. The company flags acute anomalies in real-time with its smart solutions and lessens turnaround time for improving quality.
7. Kheiron Medical Technologies (Country: UK)
The company strives to offer intelligent applications for breast cancer patients to live longer and better lives with earlier detection. It uses combined data science, deep learning, AI and radiology insights to help detect quick and earlier breast cancer detection. The company secured $22 million in 2019 from several medical giants.
8. Lunit (Country: South Korea)
It has developed AI-supported solutions for accurate diagnostic and therapeutic purposes. Lunit strives to optimize the diagnosis process at the correct cost, time, and treatment for the right patient. The company with GE Healthcare launched a chest X-ray AI analysis technique to detect and spot eight conditions, including pneumonia and tuberculosis, via algorithms.
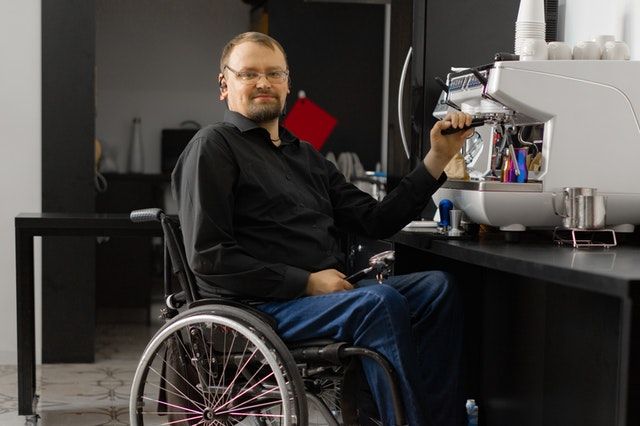
9. Nines (Country: USA)
It is one of the first and top teleradiology techniques using AI and machine learning expertise. The company is providing modern AI healthcare solutions all around the US with industry-leading results due to the support from iconic partnerships from VCs Accel Partners and 8VC.
10. Gleamer (Country: France)
A French company develops AI-based software to boost radiologists' productivity. The company gathers talent from worldwide, including engineers, AI experts, medical doctors, and managers, to create next-gen tools. Gleamer's BoneView is an exceptional medical device whose assessment can be carried out by KIWA CERMET 0476 (notified body). It provides primary data for diagnosis on standard radiography.
4. TOP FIVE APPLICATIONS OF AI IN RADIOLOGY
The first application of radiology AI was CAD (computer-aided detection), and it had a rigid way of recognition with the capability of spotting defects in the training dataset. Learning is not an autonomous process, and new skills are added to it hardcoded. AI has evolved remarkably with the potential of helping radiologists to manage different images and deploy them to connect to third-party health service providers. Here is the detail of advantages AI brings to radiology
4.1. Brain Tumors
This study can be subdivided into three essential parts: tumor detection, classifying brain tumors, and monitoring. Let's discuss it one by one.
4.1.1. Tumor Detection
Earlier tumor detection can save a patient’s life or prolong it. One of the promising technologies in AI for radiology is early detection of melanoma, as it accounts for only 1% of diagnosed skin cancers in the US but causes the maximum number of deaths. However, this aggressive cancer can be treated in five years at an earlier detection stage.
4.1.2. Classifying Brain Tumors
Nervous system cancer, including brain cancer, is one of the leading causes of death in the US. Traditionally, brain tumor patients and doctors were unaware of its type and treatment. In the first step. As much as possible, part of the infected brain mass is removed, and a sample is analyzed to classify the tumor type. This kind of pathology analysis lasts for about 40 minutes as the time taken by a pathologist for processing and staining the sample. After getting the results, the surgeon decides the course of action immediately. Luckily, AI's introduction in radiology reduces tumor-type detection time from forty to three minutes.
A recent study in the UK discovered a non-invasive way of classifying children's tumors via diffusion-weighted imaging and machine learning in radiology techniques.
In this approach, researchers use diffused water molecules and get contrast in MRI scans. After it, ADC (apparent diffusion coefficient) map is fed to the machine learning algorithm after extraction. The given technique is excellent at discovering three different types of tumors in the posterior brain part. These tumors cause a high rate of death among children. With earlier detection, the surgeon may prepare a better treatment plan and save the life.
4.1.3. Tumor Monitoring
Computer vision monitors disease spread and its bodily impact via non-invasive monitoring techniques. AI takes it one step forward by predicting the tumor throughout the treatment. It helps oncologists in detecting and predicting dynamics in real-time. REVOLVER, developed by the Institute of Cancer Research in London, is an AI-powered method to predict cancer growth. It uses machine learning to analyze cancer-causing genetic mutations. Further, extracted patterns help forecast future transformations. This technology also successfully predicts if cancer will become immune to current treatment.
4.2. Detecting Hidden Fractures
Fracture detection is another department where AI focuses on and helps treat fractures quickly, saving patients from chronic pain.
FDA started approving AI algorithms for supporting clinical decision-making in 2018. Imagen's OsteoDetect software was considered as one of the first company approved. The program deploys AI to detect distal radius fractures while scanning the wrist; the company submitted a study comprising 1000 wrist images to the FDA to support their excellent system. Twenty-four healthcare providers use the OsteoDetect tool to boost their confidence in accurately detecting fractures.
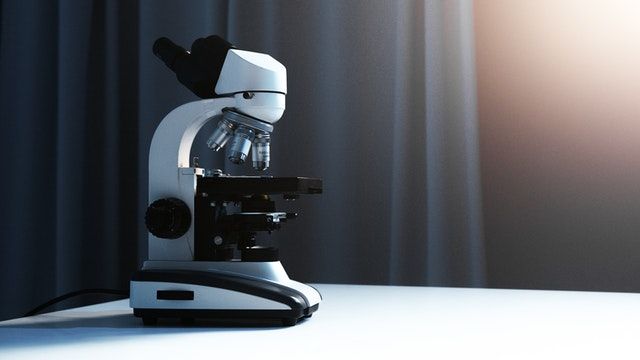
4.2.1. Spotting Invisible Fractures via AI in Radiology
Another great use of AI in radiology is detecting hip fractures, and this type of injury is mainly expected in the older generation. Before AI, radiologists used X-rays for its detection as they are hard to find because of soft tissues. In the European Journal of Radiology, a study was published regarding the potential of applying DCNN (Deep Convolutional Neural Network) to assist radiologists in spotting fractures. The technique is better to understand MRI and CT scans than the human eye. Researchers and AI worked together on analyzing the same hip fracture via MRIs and CT scans, and radiologists got an efficiency of about 83% compared to DCNN's accuracy reaching 91%.
Elbow fracture detection in adolescents and children is another example of AI applications in radiology. Cartilage is still the part of the elbow joint, making injuries unseeable on X-rays. MD Anderson Cancer Center's researchers developed an AI-powered strategy to analyze elbow joint radiographs. The technique comprises two deep learning algorithms; one involves capturing the medical image (input) and assigning weights to other parts of the image, while the other draws several patches on the image to identify patterns. The researchers tested their model on 58,817 X-ray scans of pediatric elbow images clicked at Texas Children Hospital. The accuracy obtained was exceeding 88%.
4.2.2. Vertebral Fractures
Spinal fractures are the early indication of osteoporosis and can go unnoticed as 54% of vertebral fractures remain unseen in CT scans, according to a computer scientist, Joeri Nicolaes, at USB Pharma. The researchers attempted to train a deep learning algorithm against detecting vertebral fractures and grading them. About 969 images taken from three different scanners were used to prepare the algorithm. AUC of 0.93 is achieved, making it the best fit for examination rooms.
4.3. Identifying Breast Cancer
Among US women, breast cancer is the second leading death cause, and one of the reasons is the missing up of 40% of breast lesions by doctors.
AI in breast cancer detection is an extraordinary application of AI in radiology. Teams at Google's DeepMind and Cancer Research UK Imperial Center joined their efforts to test AI's abilities in detecting breast cancer accurately. They trained the system on 76,000 images from British women and 15,000 from US women and found it an excellent tool even with the least fed information.
On the other hand, only 10% of suspicious mammograms in women are cancer-causing. It resulted in aggression, depression, and frustration that healthy women were wrongly diagnosed and treated. Radiology AI simulation tools jumped into the situation; an AI-powered tool was developed by Lunit and tested under Korean academic hospitals to aid in accurate mammography screening. They found that accuracy was enhanced to 84.8% from 75.3%. However, some women with breast cancer don't develop usual symptoms; that's why they are suggested to do mammogram screening regularly.
Complex medical imaging artificial intelligence techniques like DBT (Digital Breast Tomosynthesis) or 3D mammography make lesions visible and share remarkable details in tissue analysis. Its integration with MRI and ultrasounds enhanced accuracy with minor clinical errors. AI systems use several data points, including breast density, medical history, and risk acquiring factors, to help interpret correctly.
4.4. Neurological Abnormalities Detection
AI in radiology is excellent in detecting Alzheimer’s, ALS (amyotrophic lateral sclerosis), and Parkinson's diseases by tracking retinal movements; excitingly, it only takes 10 seconds. Speech analysis is another way of detecting Alzheimer's disease as it changes a patient's language patterns. Researchers at the Institute of Technology developed an AI tool using CNN (convolutional neural network) and trained it on healthy and suspected person texts. They found results with 95% accuracy.
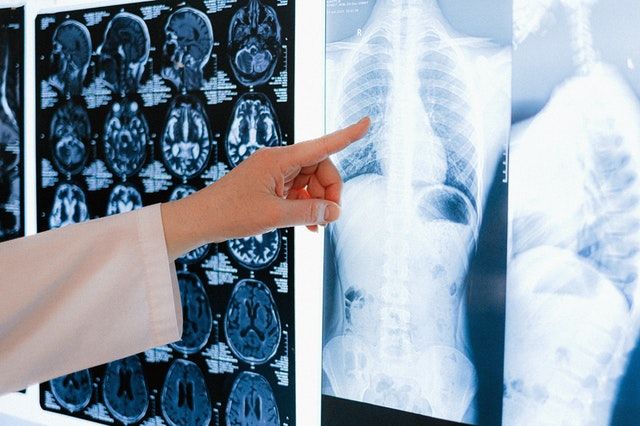
4.4.1. Neuroimaging / Brain Imaging
Neurological imaging comes with several techniques for image generation of brain structure and function or other nervous system parts. AI plays a crucial role in brain injury and trauma diagnosis. Artificial intelligence is integrated with MRI, and CT scans to get a comprehensive mapping of CNS.
4.4.2. Cardiovascular Imaging
Machine learning and trained models automated the steps involved in the complex cardiac analysis. HeartVista by RTHawk is a platform made of several advanced MRI techniques for delivering high-resolution images quickly.
4.4.3. Lung Imaging
Artificial intelligence in radiology helps successfully detect lung cancer via cancerous lesions and characteristics. Infravision, a Chinese startup, uses AI-CT (Augmented CT Screening Solution) to detect lungs lesions in CT scans.
5. AI FOR RADIOLOGISTS OFFERING SECOND OPENION FACILITY
AI algorithms running in the background offer a second opinion when radiologists have a conflict on a complex medical image. It helps reduce stress among radiologists in making the right decision at the right time. New York City's Mount Sinai Health System uses AI and human radiologists to detect COVID-19 in CT scans. The system is trained over 900 images and can notice even mild symptoms that are not detectable via human eyes.
6. AI IN RADIOLOGY BOOKS ON AMAZON
6.1. Artificial Intelligence in Medical Imaging
Author Name: Erik R. Ranschaert, Sergey Morozov, Paul R. Algra
Description: The book discusses the future opportunities, applications, and risks of utilizing this technique. It shares a deeper insight into emerging technologies on medical imaging.

6.2. AI Grid A Diagnostic Radiology Classic Textbook Essentials of Medical Imaging (in Chinese)
Author Name: LIU AI LIAN YI
Description: The book elaborated on several cardiovascular, genitourinary, and gastrointestinal systems diseases. Further, it covered females' diseases, endocrine system, clinical manifestations, nervous system, and lymphatic system. It is well equipped with unique maps and colored figures.
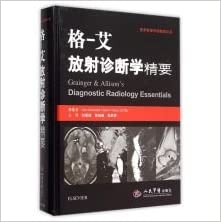
6.3. A Radiologist’s Overview of the AI Market: The First 100 Algorithms
Author Name: Ty Vachon M.D., Michael Doxey, and Kent Hall M.D.
Description: It summarizes current AI and ML possibilities in radiology with 100 FDA passed algorithms. It is ideal for medical students, residents, and radiologists to brief about past medical imaging techniques.
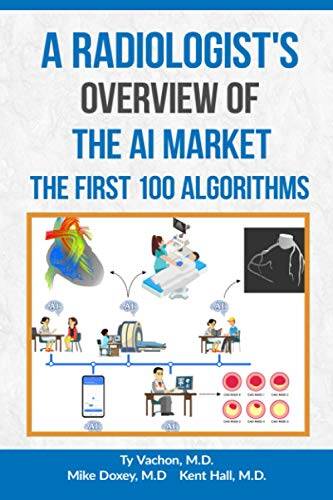
6.4. A Radiologist’s Introduction to AI and Machine Learning
Author Name: Ty Vachon and Leigh Shuman
Description: No computer background is required to understand this book. It's a short book for radiologists who want to know AI basics in radiology. It will show you that neural networks replicate the human brain while layers replace synapses.
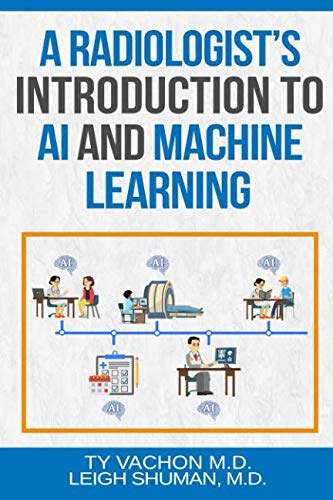
Or you may find more content on Amazon regarding radiology here.
WILL RADIOLOGISTS BE REPLACED?
Right now, AI is not powerful enough to solve all complex problems regarding medical imaging. According to Curtis Langlotz, a radiologist at Stanford, said Artificial intelligence cannot replace humans, but humans that use this technology will replace those radiologists who don't. Augmenting doctors' abilities in developing countries is another prediction for AI in radiology. For instance, Stanford University’s researchers are developing a tool that will help radiologists use their smartphones to take X-ray film pictures. The future of AI in radiology is incredible, but it is still in its maturing stages.