Robotic Process Automation - Training Time Comparison of Machines And Humans For The Same Job
To gain a competitive edge in this digital era, companies are focusing on AI-powered machines and Robotic Process Automation. However, a machine can’t accomplish a task on its own, rather it needs training just like humans, but its training method and content are different than those for humans.
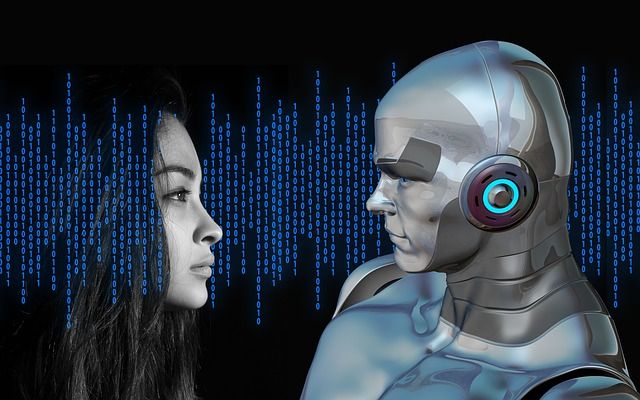
Manpower has always been considered the most fundamental asset of an organization. For decades, the intellectual property that manpower attained through continuous learning and experiences has been adding value to an organization’s growth. However, this digital era has forced ambitious organizations to focus more on highly advanced technology like AI-powered machines and RPA (Robotic Process Automation) to have a competitive edge. Today, almost every growing sector from a manufacturing company to a service provider is moving towards shifting most of its tasks from humans to machines. Tesla’s Gigafactory is over 75% automated and aims to get 100% automated, where machines would be doing all the tasks. It might not be wrong to say that out of the 7M’s (Man, Material, Machine, Money, Methods, Measurement, and Marketing), Machine, Methods, and Money, are becoming the top priority of organizations in this super-connected world. Each defeat of humans against machines is proof that machines are capable enough to replace humans in virtually every sector. However, a machine can’t accomplish a task on its own, rather it needs training just like humans, but its training method and content are different than those for humans.
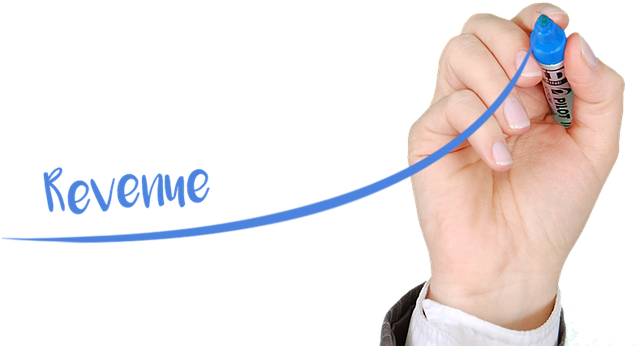
In this article, we will discuss why industries are replacing manpower with machines and how a machine becomes advantageous when compared to humans in the context of training.
Industries replacing Humans with Machines
A survey concluded that 30% of jobs in the UK are at high risk of being replaced with machines as a result of AI advancements, while 38% of jobs in the US are under threat to become obsolete by 2030. Several Startups are merging ML or DL with robotics to train the robots. Covariant founded in 2017 is using various AI approaches to train its robots. One of the most used training technique is "Reinforcement Learning" that is a trial-and-error method where a robot is given a predefined task to accomplish. Most of the machines are trained in simulations which involves an extensive training time of thousands of hours. Several other startups like Kindred, RightHand Robotics, etc., are also fusing ML with robotics.
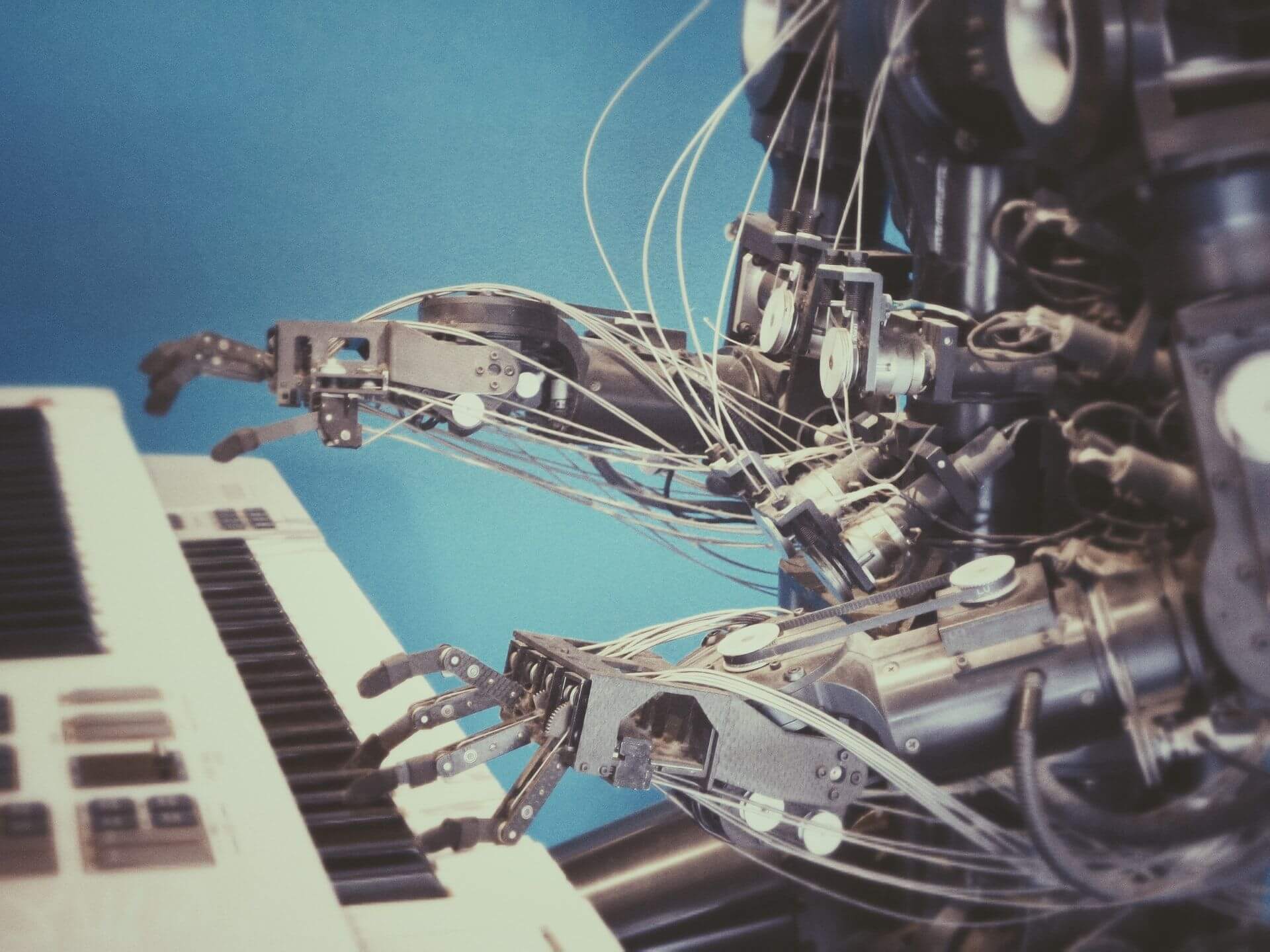
This increased focus of renowned startups on machine training is a piece of evidence that machine is soon going to become a supernatural gadget assisting organizations in a way that manpower could never do. Let’s have a look at some of the industries that have realized the potential of machines as compared to humans and are replacing manpower with machines:
Telecom Sector
Changying Precision Technology Company is a Chinese factory located in Dongguan City. Earlier, this factory was working with 650 employees producing mobile phones. Today, the factory is running with just 60 robot arms working 24/7 across 10 production lines. The company is left with only 60 workers for just monitoring production lines and computer control systems. All the remaining tasks are being handled by machines. The company claims that it has got a surprising increase of 250% in productivity and a drop in defects of 80% once it replaced 90% of its workforce with machines.
E-Commerce
In the E-Commerce industry, especially when we talk about online retailing, the main tasks include warehousing and shipping. Amazon, the e-commerce leader, is managing its warehouse with above 200,000 mobile robots. AI-powered Autonomous Mobile Robot (AMR) is trained to learn the warehouse layout and move safely avoiding warehouse obstacles. These robots transport finished products. In retail stores, robots also leverage NLP techniques for customer interactions.
Food Industry
The food industry is facing a rise in demand while a labor gap. Therefore, the industry is heavily relying on machines for increased food production and improved food quality. Machines are also used for packing, quality inspection, and managing each step of the supply chain, from harvesting in the fields to food recipes in the kitchen. By replacing human workers with robots, the food processors are observing more product consistency: i.e., same amount, weight, and packaging of each pack of a product. This task was earlier performed manually with less consistency and accuracy. The Cannabis Industry is employing weighing and packaging robots to get packaged items with more consistency. Machines do a task with more accuracy and speed far better than humans and don't get tired of being available around the clock. Furthermore, foodborne diseases epidemics are one of the major concerns in the world. Robots help in preventing cross-contamination in the food industry. Human employees can easily transmit pathogens from one place to another in the factory especially in the meat industry. Whereas, robots fixed at a place can do packaging and all other tasks perfectly without any risk of potential contamination. Robots are also washing down the factories. Machines trained on machine vision algorithms are also being used to identify the product defects in the assembly line. Such machines improve the quality of the product managing the labor gap.
Hotels
The Crown Plaza San Jose-Silicon Valley Hotel located in California is employing a robot called Dash with a touchscreen face. For instance, if a customer demands a food item, Dash will pick it up from the kitchen, put it in the locking bin, and rush to the elevator to take it to that room. Henn-a-Hotel is the world’s first Japanese hotel operated by the largest number of robots. It has replaced 90% of its workforce with machines and is delivering a great customer experience.
Restaurants
The fast-food chain is also replacing humans with machines. A robot by Miso Robotics named “Flippy” has emerged as a great human replacement during the COVID-19 Pandemic as it facilitated social distancing by removing human workers allocated at the grill during the cooking process, thereby reducing the contact of humans with food. It can grill chicken, buns, bacon, etc. Once trained, Flippy is easy to install overnight. It is trained with DL and a computer vision model. Flippy ensures a quality product through its sensors and vigilant monitoring to keep a consistent cooking temperature. White Castle, the first hamburger chain of fast food has employed the latest version of Flippy called ROAR (Robot-on-a-Rail) to its 10 kitchens. This machine has improved the production speed, efficiencies, and reduced wait times as on average it can cook 360 bins of fast food items each day. This speed of cooking is much greater than that of humans. It has a sensor bar to see in 3D, to detect the grill temperature and readiness of the burger. Flippy is also used by CaliBurgers. It is working in above 50 CaliBurger restaurants.
These are just a few examples of industries that have realized the prospective of machines and are reaping their benefits by replacing manpower.
However, to enable a machine to perform the job desired by any organization, it needs training. A machine is not a living entity that could learn on its own from its surroundings, or get a university degree as proof of its ability to be hired by any organization. Rather, extensive training is required to make a machine operational and productive for an organization. Let’s review how a machine is trained:
Machine Training
Just like humans, machines also need training before they are employed for a certain task. One thing is common, whether it is a machine or human, training involves time and patience. However, unlike humans, machines don't need to be seated in a big hall with several other fresh employees to go through the training process, and then move to another room for several days or weeks to grasp how to handle its new responsibilities. Rather machine training is a fascinating but multifaceted process.
A machine is trained through ML or DL models for data insights and automation. During training through ML, it employs ML algorithms to discover patterns. Then it modifies itself according to the data exposed, just like a child who learns from his experience. The algorithms are trained on data called “Training Data” by using statistical methods to make predictions and classifications. Hence, for training a machine through the ML model, the most crucial requirements are ML algorithms and the training data. Whereas training a machine through the DL model is a more specialized training approach that employs ANNs to imitate the human brain during data processing. With DL, the machines are trained through reinforcement, depending on continuous processing and response. DL is mostly used to train machines on technologies like image recognition, and speech recognition such as Siri, and Cortana.
Training a machine whether it is through ML or DL model, is the basic and most crucial task performed by data scientists. First of all, data is collected and datasets are prepared after filtering for high-quality data. Then an Algorithm is selected on which the model would be trained. Training of a machine involves following three steps:
- Training– it involves feeding data into the machine.
- Validation– with each cycle, the machine is trained to generate a specific prediction. Each time the factors are adjusted to ensure the accuracy of predictions throughout the training steps, then the validation data is run against that trained model to verify the algorithm.
- Testing– After passing the validation stage, the system is checked with real-world data without any labels. If the test is passed, the machine is said to be trained to perform the desired task.
How Machine Training differs from Human Training?
It might not be wrong to consider Humans as biological machines born with an astonishing level of intelligence. The in-born sensations in humans i.e., sight, smell, hearing, taste, and touch are incomparable. These sensations serve as the "Training Data" for humans to learn throughout their lives.
In the case of physical machines, the concept of inborn/innate sensory mechanism as found in humans so far resides just in the minds of sci-fi writers (even though, AI advancements are leading to develop these attributes in machines). A machine can be called a container that is filled with a blend of ML algorithms along with certain built-up learning proficiencies, yet a machine at heart. Therefore it must not be astonishing to accept that plenty of time and effort is involved to train a machine as compared to humans to perform a certain job in a specific industry or business.
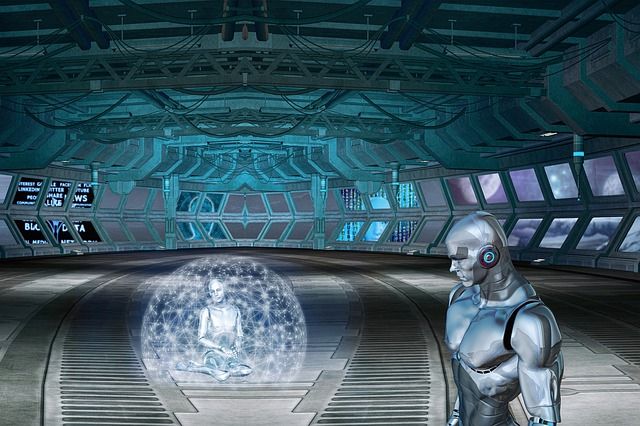
When some technology informs you about the next movie being played at your local movie theatre, it has been trained to do that task. Online searching for your requirement is not magical searching rather the algorithms have been trained to do this.
At present, the machines do all such tasks at an incredible speed with a high level of accuracy. However, a machine can't achieve this speed and accuracy on its own. Rather, it is exclusively trained to reach that level where it can perform the assigned job most efficiently. In case, a machine goes wrong, it is given an additional frequent ongoing learning that requires human involvement. Machines just like humans make mistakes. But the difference is that the humans making mistakes from birth to adulthood are taught at every step to make corrections and with time they learn from experience. Contrary, a machine is not offered the experience of 18-plus years to enable it to learn from mistakes. Secondly, they lack those innate senses to learn from the experience except that what has been taught to them through training.
While training a human, first of all, he is made aware of the purpose of learning. Then the training data is categorized into segments, and the learner is given a demonstration about how to perform that task. Then the learner is allowed to try whatever he has learned and give feedback.
While training a machine, the use case that the machine will be performing is broken down into segments. For instance, what sort of things the customer will ask, how it should respond, what info is required to answer, and where it will find the right answer to the query (i.e., through direct integration or database). After providing all this info, the machine is allowed to learn. Then a test is conducted to practice the machine to ensure that the machine is responding in the same way as expected. If not, then the training model is modified.
Hence, the approach to learning and the learning content format to train both humans and machines are different.
However, while training a machine following two things must be kept in mind:
- A machine lacks innate sensory perceptions. Therefore, high-quality data and feedback are required to train it for a specific purpose.
- The methods and the learning format used to train a machine are quite different than those for humans. The machine's understanding of the provided training must be tested. Then the machine must be allowed to practice the tasks to make sure that it meets expectations and provide feedback in a comprehensive format.
Training Time Comparison – Humans vs Machines
When it's about training, time is everything. Once an employee is hired by an organization, he is not as productive as the old employee. Generally speaking, a new employee is given training of good three months. However, the training period varies depending upon the position and infrastructure of the company. Some companies claim that a newly hired employee needs training of at least 8 months before he reaches full productivity. Employee training is very crucial as companies invest a huge amount in it each year. It is very critical to ensure that the billions of dollars are not fruitless. According to research, once the employees finish training and start routine work, they have just 1% of their work time to dedicate to their professional growth, which means just 5 minutes on daily basis. Therefore, they must fully utilize their training period to become worthwhile for the company.
While talking about the training time for a machine, it’s all about the time required to train an ML model at which the machine will run to perform a specific job. Once the model is trained, it is evaluated, and then deployed into production. In a survey, 40% of companies stated that training and deployment of an ML model take above a month. 28% said that they could do so in 8-30 days, whereas just 14% claimed that they could take just seven days or less to train and deploy a machine learning model into production. Another survey reveals that 50% of companies train and deploy ML and DL models in 8 to 90 days. Generally speaking, the training time of a machine may range from few hours to several months.
Training of a CSR and a Digital Assistant – a comparison for the same job
Customer service is a department where a company has great prospects to connect with customers. Therefore, training the customer support team is very crucial. In this modern era, customer service representatives are being replaced with AI assistants who are performing this job more efficiently than ever. Let's have a look at how the training of an AI assistant differs from the CSR training and what is the impact of that extensive machine training on performing jobs related to customer services.
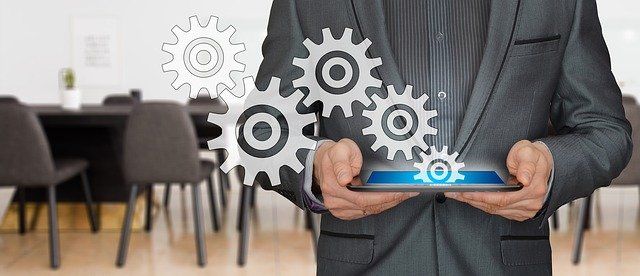
CSR– Suppose a freshly graduated student joins a team of customer service and he needs to be trained for that particular job. First of all, he will be explained what customer service is and why it is essential to respond to customer queries. Then he will be trained through demonstration about answering a query (i.e., the method to answer, the technology to use, etc.). Finally, the trainer allows the learner to practice what he has learned and provide feedback. A customer service representative is typically trained for three to four weeks.
Chatbots – These are prevailing in customer services and replacing human agents. Chatbots or automatic helplines & Digital Assistants are trained on NLP that is a field of ML. Their algorithms enable machines to identify language, comprehend it and then respond to it. In 2018, Marks & Spencer, a British retail giant replaced 100 switchboard employees with chatbots. Cortana is an AI assistant by Microsoft. To replace humans, this bot was trained extensively so that the right personality is built in its software i.e., a confident and caring personality without any bossy characteristics. Plenty of time was consumed while training these bots to demonstrate these qualities. AI assistants are nowadays trained to demonstrate even more complex human traits like “sympathy.”They promptly answer the queries related to shipping or offering personalized advice, etc. Huge datasets, ML and NLP are used in chatbots to identify text and speech inputs and to translate them. According to research, chatbots can cut short the costs of customer service by 30%. Chatbots reduce time and cost on repeated customer interactions and free up humans to focus on some creative jobs. In the case of chatbots, the complexity of the job decides the timeline. Training the latest and the most complicated chatbots take 2-3 months to be trained.
Amelia– It is an AI-powered digital assistant that has replaced humans in customer services. Amelia needs extensive training before it delivers business value. It must be integrated with all sorts of required data and must get the expertise of all processes to understand the potentially dissatisfied customers. The time to train Amelia could range from several weeks to three months, depending on the task. If it has to perform just some basic tasks, training of just a couple of weeks would be enough, however, to train it for a single-use case, a time of three months would be required. Its initial training is the most time-intensive and critical job, however once trained for a single-use case, adding other use cases for its further training is not time-intensive as the data scientist would not have to start from scratch, particularly if the use cases belong to the same industry. Amelia is extensively programmed to follow instructions with accuracy. It will never get divert from what it has been trained. Amelia is more advanced than a simple chatbot, as it is trained to understand regional accents and speech patterns. It can speak, and understand just like humans, and can perform high-level tasks with more accuracy and speed reducing the overall cost for the organization. Allstate – the US insurer is employing Amelia as a CSR.
The Cornell University Experiment 2020
Cornell University experimented in 2020 to analyze the difference in learning performance between humans and machines. In that experiment, three ML algorithms and 44 human beings participated. All of the two groups of ML algorithms and human beings were presented with training data and they had to identify patterns in that training data. Based on the pattern they find, both groups had to label instances. The results revealed that humans' performance was pretty similar across all identified patterns, whereas the performance of machines was relatively different and improved for various patterns. The experiment was conducted with 20 instances, and the results revealed that human performance did not improve with time. Contrary, machines did not learn as fast as humans but could attain the same human learning level of humans or even outperform them in 2 out of 4 patterns. However, the number of instances required to train a machine was more as compared to that of humans to produce the same results. We can say that machine training is more complex and requires huge input and time, but once a machine learns a task, it can outperform human beings in that specified task.
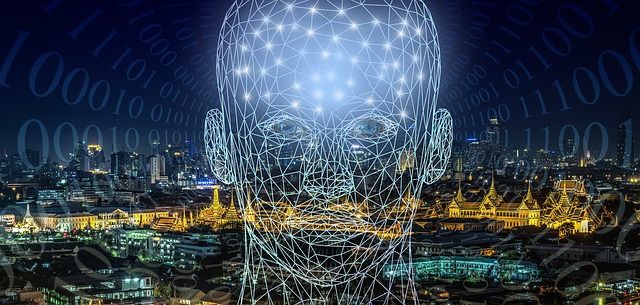
Factors affecting Training time of a Machine
There is not any obvious way to calculate or estimate the training time for a machine. It varies depending on multiple factors like hardware, the number of neural network layers, optimization, data size, and the choice of ML or DL model. The training time of a machine with a DL model is greater than that with an ML model because of increased data size. If the input data size is big, and the machine finds difficulty in handling it, then the training time will increase as everything will be slowed down. However, if a machine can handle it, then Logistic regression is the algorithm that learns the fastest. Hence a reduced training time would be required to train a machine with this algorithm.
How Training time of a Machine can be reduced?
As we have gone through before that a machine needs more complex and time-intensive training as compared to the human. But once the training of a machine is done, it can outperform humans trained for the same task. Hence, training a machine is much more advantageous than training a human. Moreover, researchers are striving hard to find ways to reduce the training time of a machine to make it even more valuable and readily available to perform a certain job. Let's discuss a few methods that could be very helpful if an organization needs to reduce the training time of machines:
Employing a Pre-trained Model – For training a machine, if an industry starts creating a DL model from scratch, then several days or weeks would be required based on huge datasets and the slow learning performance of a machine. In this case, using a pre-trained model through the process of Transfer Learning is the best approach to train a machine. In a pre-trained model, fresh data is added and the machine is trained for new outcomes. By using this approach, the data size is reduced from several million images to a few thousand; consequently, the training time is also reduced from days or weeks to minutes or hours.
Feature Extraction – A layer from the neural network that has been trained to learn a specific feature from several images is extracted. This layer with that specific feature is used in another ML model for quick machine training.
Using high-performance GPUs combined with Cloud Computing – This combination can reduce machine training time from weeks to hours. Special pieces of equipment like NVidia Tesla V100 GPU and DGX1 server are very helpful to minimize training time.
DL & ML software – Using DL software like Pytorch and Google’s TensorFlow to design a training model result in reduced training time. ML software such as Google’s Cloud AutoML and Amazon SageMaker, are also very helpful in making the whole training process much easier and convenient. You just have to select the right algorithms and context from several choices available. The rest of the work is done by a SageMaker that manages the entire core infrastructure to train that model and deploy it in the production environment. Amazon SageMaker is a well-organized service that employs the XGBoost ML algorithm to enable data scientists and developers to create, train and deploy an ML model in a short time.
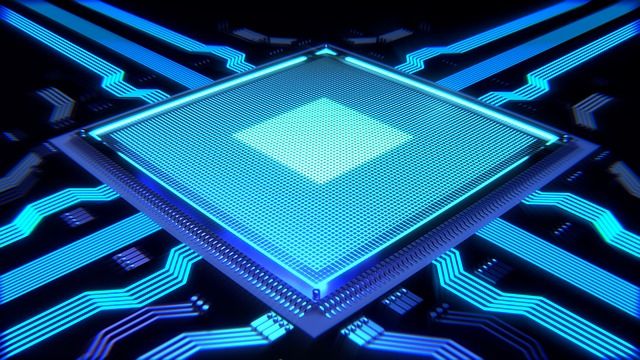
Concluding Words
In this digital era, machines are entering into each phase of human life. The automation that was just seen in movies is all set to be seen in our homes and industries. It is important to note that a machine is neither a living thing having some background knowledge from life experiences nor a magical gadget that is readily available to perform any task assigned to it. Rather, a thoughtful and comprehensive training of the machine is required that is much more complex and time-intensive as compared to human training. However, once a machine is trained, it is many times more advantageous for any organization than a trained employee. A trained machine can perform a task in a single day that a machine would complete in thousands of days. It can also perform the task of many human beings simultaneously with more accuracy. Moreover, the machines don't need salary and allowances. The expenditure just involves the acquisition and maintenance of machines. This is a single-time expense and far less than employees' monthly expenditures. That's why several industries worldwide are replacing their trained human workforce with well-trained machines to reduce operational costs and manpower and increase efficiency, accuracy, productivity, and customer satisfaction. Once a machine is fully trained to do some job, it can outperform humans.
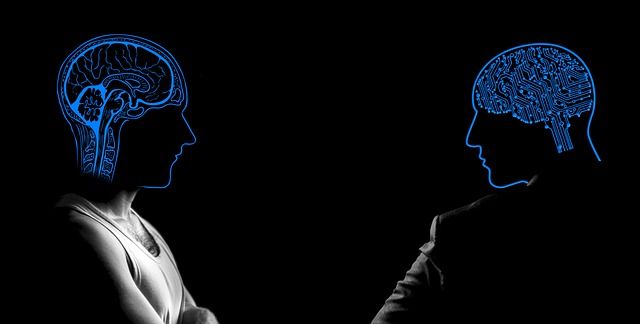