Neuromorphic Computing – the Upcoming Generation of AI
Neuromorphic computing is a recent area of study which aims to implement Artificial Intelligence in hardware. These chips are specifically designed to function like the human brain. What's so intriguing about neuromorphic computing is that it doesn't require much power to process data quickly.
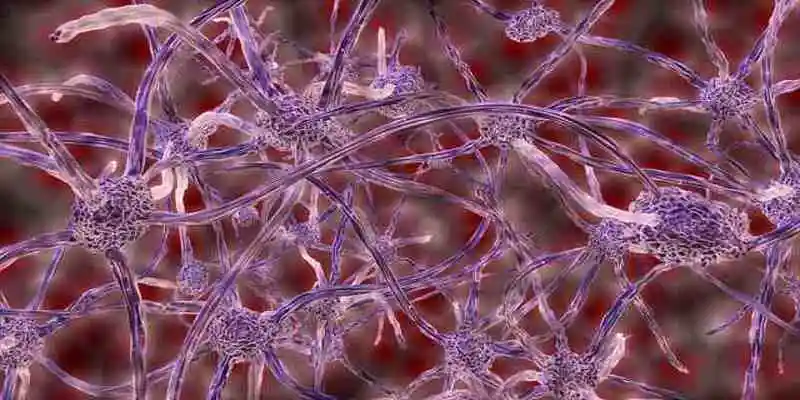
Artificial Intelligence is bringing a tsunami of change, impacting virtually every facet of our lives. From Alexa and Siri to autonomous vehicles, AI is doing miracles. Many tech enthusiasts believe that AI can usher more new life-changing developments in the entire business industry and will ultimately redefine our lives. However, despite all the achievements of AI, the human brain is still much more efficient, with less energy consumption than any AI-powered machine developed so far. Undoubtedly, today, we have sophisticated AI systems like AlphaGo that will outperform in the environment they have been trained in but will fail drastically once forced to perform in another context. This AI system uses 280 GPUs and 1,920 processing units, where each processor consumes almost 200 watts of energy. On the other hand, the human brain can process any complex task with an energy footprint of just 20 watts. So, believe it or not, it is the fact that the existing artificial intelligence is not as sophisticated as was perceived at the time of its origin in the 1950s. The reason for this gap is that the current AI is “Narrow," and its algorithms depend more or less on their training. In an attempt to reach the original vision of AI, the AI developers must create the algorithms having seemingly impossible autonomy to prevent them from being under-fitted or overfitted in a sudden unexpected situation. In simple words, these AI algorithms must not just learn but 'Adapt" the knowledge they get from their training to an entirely changed context they encounter. Truly speaking, this is the limiting aspect as AI developers can't train their algorithms for each unexpected situation they might come across. So, does this imply that Artificial Intelligence is not the technology of the future as AI experts have claimed? No, it's not like that. Luckily, we are heading towards the actual concept of AI by following the approach of “Neuromorphic Computing," where neuromorphic engineers strive to replicate the human brain in hardware. This will not just lead to an AGI system but also provide an energy-efficient path to store and process enormous data. Combined research by TU Graz Institute and Intel Lab revealed that neuromorphic hardware consumes 4 to 16 times less energy than non-neuromorphic hardware. With this enormous potential, the Neuromorphic Computing market is predicted to proliferate and reach 550,593 thousand USD from 2021-to 2026 at 89.1% CAGR.
What does Neuromorphic Computation mean, and How does it Work?
Neuromorphic Computation is a branch of AI that leverages the functioning of AI-powered devices. The term, first coined in 1980, refers to developing a computer engineering model on the pattern of the human brain. It follows the principles of neurobiological Computation and employs the latest algorithmic approaches to replicate the interaction of the human brain with its surroundings. Spiking Neural Networks (SNNs) that excite natural learning are used in this Computation. These SNNs aggressively re-map neural networks to enable them to learn by themselves from the patterns and respond in any situation based on their learning. These event-based asynchronous SNNs assist neuromorphic processors in becoming more powerful and well-functioning than conventional architecture.
The neuromorphic engineers design the neuromorphic hardware (Chips) that combine memory and processing in one unit, just like the human brain. These chips serve as neurons and can communicate and interact with each other. They aim to build devices that would be capable enough to learn, memorize information they receive, and think to represent the aptitude that resembles human cognition. Researchers are leveraging neuroscience to develop a human brain through this approach artificially. It might sound insane and bogus, but the researchers consider it the roadmap to achieving Artificial General Intelligence – the True AI.
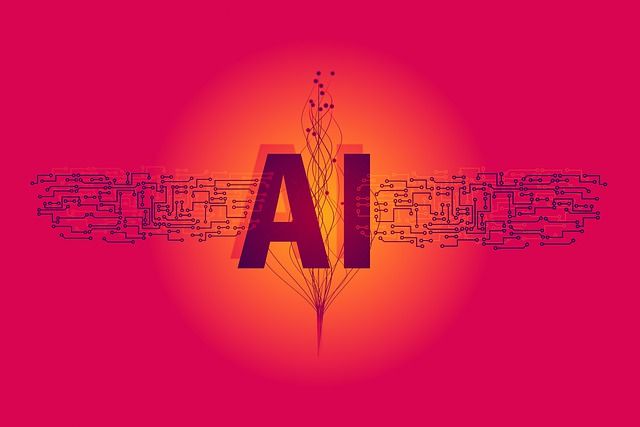
What Brings the Need for Neuromorphic Computing?
1. ANNs are not Truly Imitating the Human Brain
The question might be striking: when ANNs already mimic the human brain, what more can Neuromorphic Computing bring? What is the purpose behind it? Let me give you the answer to this!
Historically, AI experts have tried to mimic how the human brain works, and introduced the model of ANNs (Artificial Neural Networks), which has been implemented in numerous AI-powered devices. Unfortunately, it’s just software-based deception and lacks the main principles of the human brain that enable it to respond to any unexpected situation quickly.
Although the modern ANN is inspired by the human brain's neural network, it can't be said the true Replication as both are not too close in similarities. This conventional approach of ANNs needs to be upgraded with the progression of “Neuromorphic Computing," where the conventional architecture is re-engineered as SNNs (Spiking Neural Networks). This advanced architectural approach will augment the autonomy of AI systems and help accomplish its original goal – Artificial General Intelligence.
2. AI needs an Incredible Hardware to Store Gigantic Data
With the advancement in technology, the amount of generated data is increasing rapidly. The modern processing units consume massive energy while processing this vast data. Today we are at a point where Moore’s Law is moving towards its end that would ultimately stagnate the performance of our modern computers. Therefore, besides new algorithms, AI needs special hardware that can adequately store and classify data. To counterpart the upcoming situation, Neuromorphic engineers have designed brain-inspired "Neuromorphic Chips." These chips can store ever-increasing data and process it much faster with reduced energy footprints.
Intel – Leading the Way to Achieve “True AI”
Intel – a US-based tech giant, has already taken a step towards achieving “True AI” by inventing a chip that replicates the human brain. This prototype chip launched in 2017 is called “Loihi" and can perceive, learn and understand as the human brain does. It has 1024 spiking neurons and a memory of 208 kB. Intel also aims to make these chips accessible as a cloud service.
Loihi 2 – It is the second generation of Neuromorphic Chip that Intel introduced in 2021. Its processing is up to 10 times faster than its first-generation “Loihi ."It has about 15 times more resource density with lesser energy consumption.
Intel has plans to integrate its neuromorphic hardware technology into a CPU and introduce a “Neuromorphic Computer” to the world. This new technology will augment the system with an energy-saving AI processor.
Neuromorphic Computer – A Revolution in the World of Supercomputers
Today's computers possess more incredible memory, storage, speed, and processing power than ever. They are working and improving amazingly, then why do we need a revolution?
Neuromorphic Computer, as the name indicates, employs a computer model inspired by the human brain’s functionality. The human brain serves as a more tempting computing model than supercomputers. The brain is more compact and needs less energy than a supercomputer. A human brain needs 20 watts, while the Fugaku Supercomputer – one of the most powerful supercomputers worldwide – uses 30-40 megawatts. Unlike the enormous cooling system for supercomputers, the human brain resides in a bony housing to maintain it at 37oC. Therefore, computer giants are planning to use Neuromorphic hardware to develop a Neuromorphic Computer. This system will work far more similarly to the human brain.
Neuromorphic hardware has two incredible features of the human brain that lack in ANN or any conventional CPU architecture.
- Parallelism
- Asynchronism
1. Parallelism
The human brain comprises millions of neurons that function according to the instructions they receive from their local environment. They don't need to wait for the memory pool to send instructions for what to do next. Similarly, a neuromorphic processor comprises a network of several parallel units with fixed allocation memory and computational capability within each department. There is no separate system for memory and processor as with traditional CPU architecture. Hence, it removes the Von Neumann bottleneck that refers to the throughput limitation of a computing system. It involves the time in which data leaves the device memory and reaches the processing unit. In conventional CPU architecture, the processing unit must wait until it gets the data. In the case of neuromorphic chips, the entire computation process occurs in the system's memory, so it does not involve any bottleneck. Memristor – an electronic memory device is the base of these chips and is considered the first inorganic neuron, serving as memory and computational units.
Just like the human brain, which can work on millions of calculations simultaneously, neuromorphic hardware is highly parallelized, improving the training and response speed of neural networks.
2. Asynchronism
A traditional processing unit has to execute a series of instructions individually until done with all. Therefore, the execution speed depends on the processor’s clock speed expressed in GHz. Contrary to this, the human brain lacks such a clock. Here, the thoughts occur when an electronic impulse triggers the neurons. This is the same model neuromorphic hardware follows. Everything that befalls in the neuromorphic hardware results from a stimulus (called a neural spike) that occurs first to trigger neurons. Therefore, it is called Asynchronous (event-based) instead of Synchronous (clock-based).
How Far are We from Developing Neuromorphic Computers?
Developing the Loihi and other neuromorphic chips alone does not verify that we are about to develop Neuromorphic Computers. We are still far away from creating an artificial human brain. The most powerful chip to date is the “Loihi 2 Chip," and it can boast just one million neurons, whereas the human brain resides with 100 billion neurons. Undoubtedly, tech giants like Intel and IBM are striving hard to elevate that architecture but believe that it's not an easy task to develop software for it. In an attempt to get closer, Intel introduced Lava – an open-source framework to help neuromorphic engineers develop apps for Loihi.
Challenges
1. Poor Visibility
If you were unfamiliar with Neuromorphic Computing before reading this write-up, don't worry, as you are not alone. Though the concept is not new, the researchers started deliberately working on it a couple of years back. But the working of neuromorphic systems is so complex that grasping them is a challenge. AI engineers might be aware of this concept, but they are just applying the conventional approaches to AI algorithms. Hence, improving the visibility of neuromorphic Computing is the first and foremost challenge that requires several creative minds to push it.
2. Human Brain Imitation is a tall order
Developing an artificial human brain is an arduous and complex job. Until now, we have not precisely worked out how the human brain functions, so extending its imitation could be tricky with missing puzzle elements. Neuroscientists have undoubtedly improved our understanding of our brains; but there are still many unsolved mysteries.
3. A Drastic Change in Computing Norms
Neuromorphic Computing is associated with dramatic changes as it will essentially revamp the way we currently acknowledge computing norms. Instead of observing the von Neumann model, this new approach will commence its own norms. For instance, the modern computers we use today perceive an image as a series of individual frames using the von Neumann model. In contrast, the Neuromorphic Computer would ignore this notion supporting encoded information and will follow its own standards, about which even the humans might be unaware.
Neuromorphic Computing will demand brand new machine codes and frameworks. They will need even more storage capacity, substantial memory, and powerful sensory devices to benefit from the new architecture. The new connection between processing and memory will also change the development and implementation of the devices that involve those processes. This is going to be a paradigm shift, but we are yet at its nascent phase.
Why is Neuromorphic Computing the Future of AI?
With all the reality checks, AI experts believe that Neuromorphic Computation is the only road that would lead us to achieve AI in its real sense.
The traditional processors running Artificial Neural Networks have high energy consumption and latency as they have to wait for the instructions to be received from the memory. Neuromorphic chips, being more similar to the human brain, have memory and processor in the same environment leading to a much higher speed. The conventional hardware (CPUs and GPUs) needs hundreds of watts to execute information, while a neuromorphic chip like Loihi mostly consumes less than one watt as the neural spikes are scarce in time. All the neurons in a neuromorphic system are not active every time. Therefore, one SNN can replace hundreds of traditional ANNs. Hence, the output from each neuron in SNN is much more than that of an ANN. It eventually improves the efficacy of neuromorphic hardware. The parallel and asynchronous architecture also enables to respond to any input promptly. This low latency and energy-efficient feature make neuromorphic Computing more suitable for AI-powered devices. Because the Neuromorphic engineers don't aim to develop AI algorithms to analyze big data sets; instead are prone to creating AI algorithms that could learn and think just like humans. They are basically replacing the synchronous processing of the fundamental computing engines – CPU and GPU with event-based asynchronous Computation of the human brain that will allow industries to develop extremely fast AI solutions.
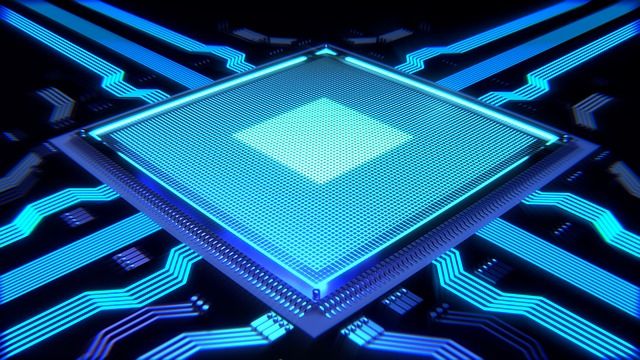
Neuromorphic Technology will Improve the Autonomy of AI-Powered Devices
- Autonomous cars – one of the most amazing inventions of AI, imply mainly 4 or 5 G technology and neural networks. It links with a data center that receives the data from the car, then analyses it, and sends it back to the vehicle via 4/5G technology. It takes time which may produce latency resulting in the loss of human lives. If the autonomous car is based on neuromorphic technology, the entire processing will occur locally, i.e., inside the car's neuromorphic chip (brain). It will result in reduced energy consumption, latency, and increased autonomy of the car.
- Smartphones – According to Samir Kumar- director at the research lab of Qualcomm, the neuromorphic chips once fixed in smartphones, will enable them continuously monitor your activities and location to figure out when and where you could need it, and it will offer you the help in advance to come out of a problematic situation.
Neuromorphic Computing will lead AI to penetrate Metaverse
With every passing day, we are moving towards the Metaverse – the virtual world where the systems will be carrying out billions of transactions simultaneously. Metaverse- that will not simply be a place where we will see avatar-wearing humans communicating and dealing with other similar humans in a digital 3D space, but rather a world where intelligent artificial agents would exhibit human behavior. So, Neuromorphic Computing is a perfect fit for it as it will power AI to permeate deeply into the Metaverse.
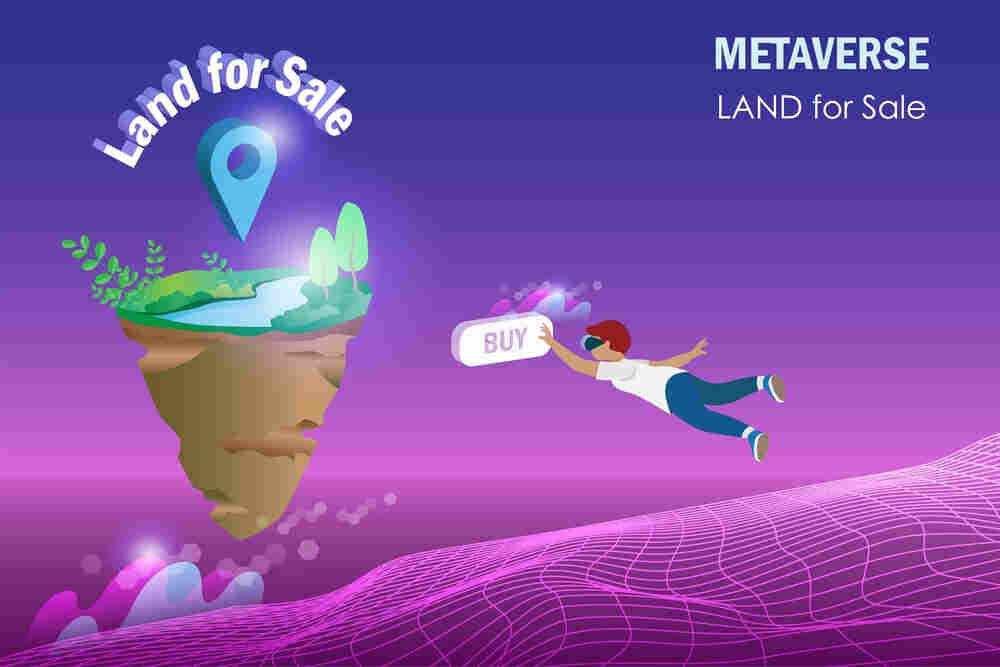
In a nutshell, AI is becoming faster, improved, and more general with Neuromorphic Computing. This approach is fascinating as it will lead to tremendously intelligent applications and ultimately supersede human intelligence.
Final Thoughts
While the intelligent machines and robots we have today exhibit the potential to transform our lives, Neuromorphic Computing puts forward an entirely new way for us. It can be said the real future of AI as it aims to accomplish all AI-related dreams. With the advancements in Neuromorphic Computing, we are heading towards achieving the goal of “Artificial General Intelligence," where AI systems would be indistinguishable from human beings. So, it would not be wrong to state that Neuromorphic devices are the upcoming generation of AI systems capable of possessing cognitive behavior just like humans.
Brad Aimone – a neuroscientist and researcher at Sandia National Laboratory, says: