Top 5 Cost-Cutting Artificial Intelligence (AI) Use Cases in Banking and Finance
Artificial Intelligence (AI) can be used in financial sector especially in Banking to reduce and optimize cost of Operations, Customer Acquisition, Merchant Acquisition, Advertisement and Marketing, Sales and Human Resource. This is an era of Digital Transformation, Disruptive Technologies, Fintech
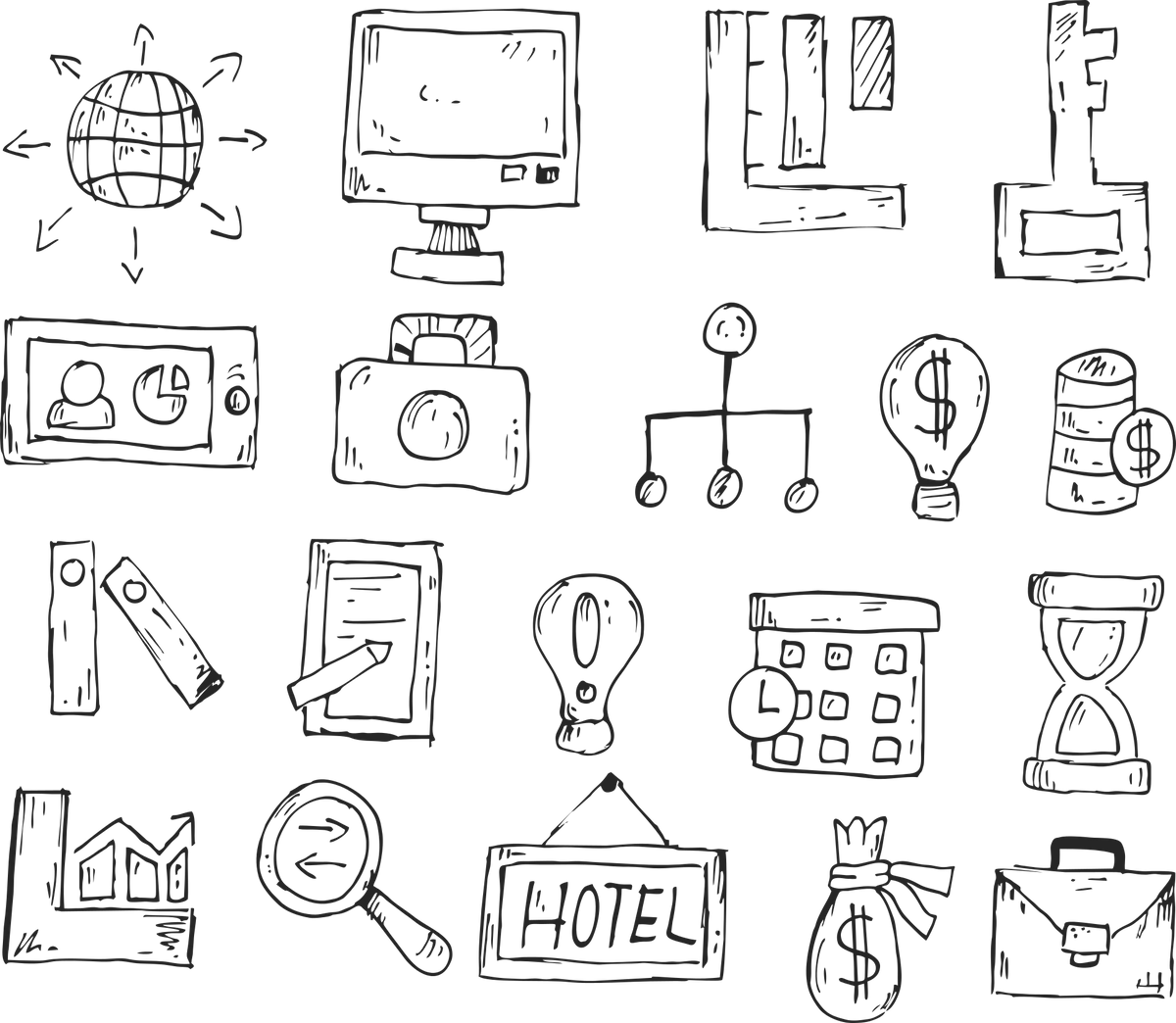
Artificial Intelligence (AI) can be used in financial sector especially in Banking to reduce and optimize cost of Operations, Customer Acquisition, Merchant Acquisition, Advertisement and Marketing, Sales and Human Resource. This is an era of Digital Transformation, disruptive technologies and Fintech where banks are going digital and AI is playing a vital role to make work seamless, intelligent and automated. Artificial Intelligence means giving brainy work to machines and computers. The current era is focused on AI and its marvelous manifestations. Artificial Intelligence is changing our lives day by day. From health to entertainment, and from packing to autonomous cars, every field is supported by Artificial Intelligence. Finance is the second most crucial aspect of human life and it is also aided by automation.
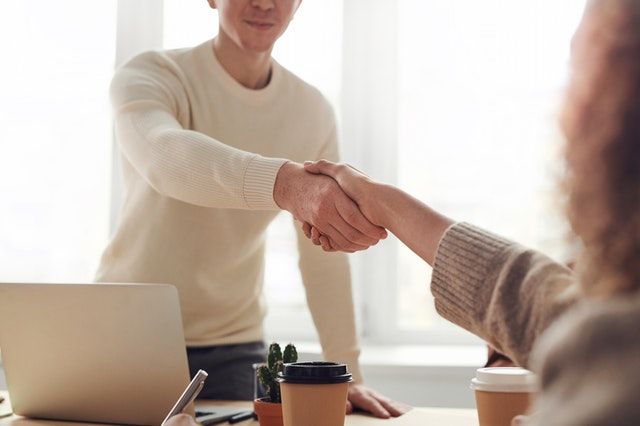
Traditional banking requires a building, staff, and hell lot of data to be maintained on ledgers or computers now. With the swiftness of every other aspect of life, human wants banking to be swifter. The time of standing in the long queues in the front office of a bank is coming to an end. Artificial Intelligence is cleaning the way.
The basic question is, “Why we need to incorporate AI into banking? Just for being talk of the town or a buzzword?” The answer is “No!” With the increase in capital marketing and innovations in business, the bank should adopt more rapid and flawless techniques to handle, store, and execute the financial data.
Also read this Top 10 AI Trends in Banking
Here are key points about why banking should be aided by AI:
- Speed and accuracy for banking operations
- Operational cost reduction for a better return on equity
- Expanded time of banking hours
With all the above in mind, let’s discuss how AI is helping the financial sector to reduce and optimize costs and improving operation.
The below list of use cases is prepared by evaluating two main aspects:
- Reduce operational cost
- Increase banking revenue
1) Chatbots and Digital Banking
Keeping in mind the factors where a bank has to pay its expenses, the first and foremost thing is presence of physical offices. The cost of running a physical office includes buying/renting the building, power consumption cost, and last but not least, salaries of the human workforce.
Whenever a bank tries to cut the expenditure, naturally it thinks about the human workforce. This may not be a tyranny because sometimes a human representative cannot perform all the front office functions including dealing with cash deposits and withdrawals, guiding customers about bank’s policies and offers. Especially when there is a rush in the bank, the human mind gets confused to increase the chances of error ending in an unequal balance sheet. So this results in delayed operations and financial loss to the bank.
Due to advancements in AI, many banks have deployed AI-supported Chatbots to mimic the human representative. People often are unable to distinguish between a human and a chatbot while communicating through online chat. Plus point of using chatbots is that banks can operate for an extended time without giving any over-time i.e 24/7/365. Another positive aspect is that chatbots can handle multiple clients at the same time with the same accuracy that is encoded in the program.
Example of a Bank without a physical location
Ally is a 100% online bank without any physical office on earth. The total revenue of Ally Financial until 2019 was US$6.394 billion with a net income of US$1.721 billion. Besides common banking operations, Ally offers Self-Directed Trading, Managed portfolios for automated investing, and even home loans.
By taking all operations online, banks can save the cost of the physical building, energy consumption charges, and human workforce salaries. This enables a bank to focus more on improved and complex banking operations.
Similarly, Discover Bank is another example that does not have physical branches or offices.
2) Minimize Errors Afflicted by Human Workforce
AI can help to minimize errors afflicted by the human workforce. Machines are emotionless and tireless entities that do not bother the weather, burden of work, or emotions. If carefully programmed, bots and AI software can perform labor extensive and sensitive tasks without any error. A big loss can be inflicted on the banks if records are not managed carefully. 38% of banking losses are due to human error. This reduction in revenue must be paid by the bank and it is considered as “Loss”. If a bank can minimize the loss induced by miscalculations and human error, it can increase its net income.
Examples of AI solution used for banking audit
OSP has designed a customized “AI in Auditing Solutions” program that works faster and smarter for internal audits. Their intelligent auditing assistant performs chunks of tasks that are crucial for audit. These tasks are AI-driven Audit reporting, Audit planning systems, Role-Based Access Control, intelligent data sampling, and Journal Entry Testing.
Another interesting tool is offered by HighRadius named “Bank Reconciliation Cloud”. It audits the daily closure by automatic classification of bank transactions into operational and non-operational cash categories. The best thing about this program is it is a plug n play system with integrations with multiple ERP systems like SAP, Oracle, MS Dynamics, etc.
3) Formulate Personalized Offerings
AI can aid the banks to formulate personalized offerings. Banks deal with a wide range of customers i.e. from individual to enterprise. The most prevalent practice to generate bank income is to do business. After The Great Recession (Dec 2007 to Jun 2009), Fintech (financial technology) emerged. With the emergence of Fintech, traditional banking has been considered a “slow-train”. This new technique is quite disruptive, forcing traditional banks to evolve into large fintech organizations.
Traditional banks are losing their small customers as the investment capabilities of an individual are shrinking. COVID pandemic also proved to be “Icing on the cake”. For the sake of cost-cutting in baking, a bank may thoughts of increasing trades and revenue without increasing operation cost for extra slots. The easiest solution to solve this problem is to introduce more intriguing personalized offerings. By analyzing the customer’s purchase, saving, and financial attributes, a bank can design the personalized offering that can attract the individual/ enterprise clients more vigorously.
The main issue is that a bank may have hundreds of thousands of customers and preparing customized single personalized offerings is a laborious and expensive approach. But thanks to the latest technology, banks now can utilize the power of artificial intelligence to analyze each customer’s financial behavior. But there is one problem. If a bank is unable to program its algorithm for robust personalized offerings, then there could be a challenge to outsourcing this agenda to third parties. Thanks to the UK’s Open Banking initiative and the European Union’s Payment Services Directive 2 that allow wide access to customer’s data.
AI-based solutions for personalized offerings in Banking
Few names offer support to the banks in pursuit of data-driven personalized offerings. CrayOnData is one of the examples that aid banks to design personalized offerings platforms. Besides this, Optimizely and Maya AI are also major contributors in this regard.
4) Mandate and Compliance
AI can help with Regulatory Compliance. Every bank wants to enhance its profits but these desires cannot go unleashed. There are laws and regulations for banking, failing to follow which can result in legal punishment and federal fines or penalties. This also increases the banking cost and this issue must be dealt with cautions. Regulatory compliance is a practice for following all the laws.
Keeping harmony with ever-changing banking laws is a hard nut. A highly skilled team is required to maintain Regulatory Compliance. This results in potential human error and high cost under the head of salaries. Humans can never assure 100% accuracy of analysis or policymaking. But if regulatory compliance is practiced based on previous data, more insights can be observed and faster decision making can be achieved.
Artificial Intelligence is destined to serve even in this complicated and accuracy demanding field. AI and machine learning can be deployed to read the previous compliance data and it can help compliance officers dealing with daily policy challenges.
AI can aid in Regulatory Compliance by promising three-factor output i.e. reducing false positives, lowering costs, and determining human error.
False-positive reduction using AI
The compliance division faces false positive on daily basis in hundreds or thousands of counts. False-positive generates an alarm and then it is reviewed by the compliance team. AI and ML are quite apt for capturing, analyzing, and filtering huge data elements in no time and with high accuracy.
AI can reduce the cost of regulatory compliance
If compliance is carried out by AI-driven software then there will be no need for highly skilled staff that usually demands 6 figure annual salaries. Plus, AI systems never get tired or bored and they can perform 24 hours duty. Intelligent systems are trained in such a way that each new regulation can be intercepted in a much faster and more accurate way.
AI and ML are addressing human error in regulatory compliance
The AI-controlled machines can never defy the law of coding. It will perform the required coded function without any error. Human error can cost the banks millions of dollars if analysis or calculations are erroneous. Companies mostly acquire AI where human error cannot be tolerated.
The above parameters are supported by AI-based platforms including ComplianceAI’s RegTech, MindBrigeAI, and Ascent RegTech. Among these, MindBridge has won Central Banking Fintech RegTech Global Awards in 2018.
5) Capital Optimization with AI and ML
Capital Optimization is a vital prospect of any banking system. It is defined as optimizing the balance between payables and receivables. Since assets are less liquid, so Capital Optimization mainly focuses on Cash-On hand and any other with high liquidity that can be converted into cash within 90 days.
Working capital is the backbone of every bank. In the time of recession or uncertainty, the most precious treasure of a bank is capital and it must be saved through proper optimization without ceasing or minimizing the banking operations. Reducing cash flow is an emergency solution but not for the long term. Supply chain and financial workflows must be intelligently optimized for greater and long-lasting advantages.
Cash flow records are not easily maintained, they are usually in million numbers. Manual record-keeping is not a good practice. Thank data-driven AI and ML that the most ancient and tinniest cash boxes are no longer hidden or overlooked. Artificial intelligence can make things happen in a much faster and accurate way. A small training set is provided to the AI platform and then these platform scans are the data from the data repository i.e. cloud server or local storage.
How Capital Optimization is processed
There are two approached to cope with the task.
- Quick approach
- Long term Approach
The quick approach usually spans over four weeks and can be designated as an emergency measure to reduce cash outflow by
- Inhibiting readily payments
- Avoiding Non-Profit Expenditure (NPE)
- Calculating surplus stock to avoid an unused stack of goods
- Optimizing cash return terms and methods
Long Term approach is more like a permanent solution of optimizing working cash flow. This approach relies on the following practices:
- Maintaining supply chain accuracy
- Expenditure tracking
- Optimizing assets and liabilities
- Fine-tuning the transaction processes
The leading platforms for AI-based cash flow optimization are Deloitte, HighRadiusAI-powered cash forecasting platform, and Celonis.
Ending Note
Cost-cutting for financial and banking sector is not an easy task as it seems. The factors for running a bank are enormous. Without taking care of every aspect, no bank can achieve excellence. Artificial Intelligence gave another life to the dying banking system. Automation and improvement are direly needed more than ever before. As Palo Alto says, “Digital Darwinism is unkind to those who wait.”
Cheers :)